Quantitative Biology
The Simons Center for Quantitative Biology (SCQB) is Cold Spring Harbor Laboratory’s home for mathematical, computational, and quantitative experimental research.
Research in the SCQB centers on genomics (how genomes work, how they evolve, and what makes them go wrong in disease) and neuroscience (how brains are structured and how they process information). In addition to advancing biological knowledge, members of the SCQB develop broadly useful experimental, computational, and mathematical methods for the wider research community. BioAI—the interface of cutting-edge biological research and revolutionary artificial intelligence technologies—is a major theme of much of this work.
The SCQB is supported by a generous endowment from the Simons Foundation. Additional funding has been provided by the Starr Foundation and Lavinia and Landon Clay.
Our faculty are experts in the mathematical and physical sciences who address open problems in biology, often in close collaboration with experimentalists. Most research in the center falls in the general areas of gene regulation, evolutionary genomics, disease-related human genomics, and genomic technology development. However, our work also touches on neuroscience, immunology, and plant biology, among other fields.
Members of the SCQB maintain close collaborative ties across CSHL and with many other New York area groups, including Stony Brook University and the New York Genome Center.
Leadership
Interim Chair
Justin Kinney, Ph.D.
QB/AI Seminar Series Lead
Hannah Meyer, Ph.D.
Center Staff
Sr. Scientific Administrator & Assistant to the Chair
Susan Fredricks
Scientific Administrator
Antonia Little
Assistant Director of Administration, Cancer & Simons Centers
Katie Brenner
Quantitative Biology External Advisory Committee
This Simons Center for Quantitative Biology External Advisory Committee meets annually to provide strategic advice and general guidance.
Andrew G. Clark, Ph.D.
Professor of Molecular Biology and Genetics
Cornell University
David L. Donoho, Ph.D.
Anne T. and Robert M. Bass Professor of Humanities and Sciences
Professor of Statistics
Stanford University
Eric D. Siggia, Ph.D.
Viola Ward Brinning and Elbert Calhoun Brinning Professor
Head of Laboratory of Theoretical Condensed Matter Physics
The Rockefeller University
Steven L. Salzberg, Ph.D. (Chair)
Bloomberg Distinguished Professor of Biomedical Engineering, Computer Science, and Biostatistics
Director, Center for Computational Biology
Johns Hopkins University
Simons Center for Quantitative Biology Annual Reports
-
- 2024 Annual Report (PDF)
- 2023 Annual Report (PDF)
- 2022 Annual Report (PDF)
- 2021 Annual Report (PDF)
- 2020 Annual Report (PDF)
- 2019 Annual Report (PDF)
- 2018 Annual Report (PDF)
In nature’s math, freedoms are fundamental
May 28, 2025
CSHL quantitative biologists have developed a unified theory that could have countless applications, from plant breeding to drug discovery.
At the Lab: AI evolves
May 20, 2025
CSHL neuroscientists discuss the biological brain’s multibillion-year advantage over AI—and the new algorithm they built based on that concept.
The CSHL School of Biological Sciences’ class of 2025
May 5, 2025
The School of Biological Sciences awarded Ph.D. degrees to nine students this year. Read some of their stories and reflections on their time at CSHL.
A perception quiz to blow your mind
March 26, 2025
Ready to think differently about how you see, smell, hear, taste, touch, and make sense of the world around you?
The greatest challenge in the universe
March 17, 2025
How does the brain turn sensory information into world-building neural responses? New answers could open the door to AI-powered therapeutics.
PSEG Long Island awards CSHL $279K in energy rebates
January 22, 2025
Cold Spring Harbor Laboratory’s sustainability initiatives reduce the institution’s operational costs while empowering high-tech research.
The 2024 CSHL Volleyball Final
December 24, 2024
Eight teams entered the season on equal footing. Now, only two remain. But there can be only one champion. Press play to watch it all unfold.
Navlakha named Simons Foundation Pivot fellow
December 5, 2024
The computational biologist teams with CSHL’s Hannah Meyer to explore how the immune system solves problems also common in AI.
The next evolution of AI begins with ours
November 25, 2024
How do innate abilities get passed down? Cold Spring Harbor neuroscientists have devised a solution that could lead to faster artificial intelligence.
Empowering Insights: The science behind health
November 18, 2024
“The opportunity to turn curiosity into discoveries that impact the human condition is at the core of CSHL’s mission,” writes President Stillman.
At the Lab Season 1 Research Rewind: AI+
October 29, 2024
This season’s final Research Rewind brings us from the realm of quantitative biology to neuroscience, genomics, and beyond.
At the Lab Season 1 Research Rewind: Genetics
October 22, 2024
It’s the code for all life on Earth. This week At the Lab, we’re hacking it with the help of Cold Spring Harbor Laboratory’s geneticists.
Great minds think AI
October 16, 2024
Innovators and thought leaders in the fields of artificial intelligence and neuroscience came together for a meeting at Cold Spring Harbor Laboratory.
At the Lab Season 1 Research Rewind: Neuroscience
October 15, 2024
What do you think? How do you know? And who are you anyway? We probe each of these questions with the help of Cold Spring Harbor’s neuroscientists.
NeuroAI with an eye on equity
September 30, 2024
Working at the intersection of neuroscience and computer science, CSHL researchers aim to build AI that will benefit everyone, not just the lucky few.
How does cancer spread? Follow the map
September 25, 2024
CSHL Professor Adam Siepel and postdoc Armin Scheben use genetic barcodes to map how prostate cancer spreads.
The curious immune cells caught between worlds
September 24, 2024
CSHL’s Hannah Meyer shows innate-like T cells mature differently in humans and mice. Her discovery could improve preclinical immunotherapy studies.
CSHL grad student wins International Birnstiel Award
September 23, 2024
Shushan Toneyan won the award for her thesis research in CSHL’s Koo lab. Toneyan is the co-creator of CREME, an AI-powered virtual laboratory.
Is CREME AI’s answer to CRISPR?
September 16, 2024
CREME, the latest AI toolkit from CSHL, is a virtual laboratory that may help scientists find new therapeutic targets in the genome.
Making headlines
September 11, 2024
Several Cold Spring Harbor Laboratory faculty members received national mainstream media attention in 2024.
The nervous system’s matchmaker
September 2, 2024
CSHL’s Saket Navlakha has devised a new computer algorithm that could have many popular real-world applications. His inspiration: the nervous system.
At the Lab Episode 17: AI SQUID
July 30, 2024
Tune in to this week’s podcast to hear about the latest artificial intelligence model coming out of Cold Spring Harbor Laboratory.
At the Lab Episode 15: Fruit flies’ dating lives
July 16, 2024
Can AI help us identify the Don Juan of fruit flies? CSHL’s Benjamin Cowley thinks so. Why would we want to do this? Tune in to find out.
At the Lab Episode 14: What’s that smell?
July 9, 2024
You might not realize, but that question is central to the human experience. On this week’s podcast, CSHL’s Saket Navlakha sniffs out answers.
SQUID pries open AI black box
June 21, 2024
CSHL’s Koo and Kinney labs have built a tool to suss out how AI analyzes the genome. What sets it apart? Decades of quantitative genetics knowledge.
New AI accurately predicts fly behavior
May 22, 2024
CSHL’s Benjamin Cowley trained an artificial intelligence model to predict how real-life fruit flies will act in response to specific visual stimuli.
At the Lab Episode 8: Birds of a feather
May 21, 2024
How did some birds get such distinct colors? CSHL Professor Adam Siepel joins us for a journey across evolution’s “islands of differentiation.”
Autism genetics: The faces behind the data
May 16, 2024
CSHL research on autism involves massive databases with thousands of genomes. Meet a few of the brave individuals who help make this work possible.
The CSHL School of Biological Sciences’ class of 2024
May 5, 2024
The School of Biological Sciences awarded Ph.D. degrees to 11 students this year. Here are some stories and reflections from their time at CSHL.
At the Lab Episode 5: A heart of golf
April 30, 2024
A 500-year-old mystery stumbled on by Leonardo da Vinci has been solved using modern clinical data. Meet the CSHL scientist at the heart of it all.
David Klindt joins CSHL neuroAI team
April 25, 2024
The new assistant professor will study how our brains navigate a continuously changing sensory environment: His goal: build more sensible AI.
Cocktails & Chromosomes: Through the eyes of a fruit fly
April 18, 2024
CSHL Assistant Professor Benjamin Cowley takes us inside the mind’s eye, using an AI model of this tiny insect’s brain.
Mitra Javadzadeh joins CSHL neuroscience faculty
April 18, 2024
Javadzadeh is the newest Cold Spring Harbor Laboratory fellow. Her lab studies how the brain processes visual information.
CSHL goes to the White House for Easter EGGucation
April 8, 2024
CSHL plant scientists taught kids the basics of plant biology and its role in the environment at an event hosted by First Lady Jill Biden.
Why some RNA drugs work better than others
March 6, 2024
CSHL’s Justin Kinney and Spinraza inventor Adrian Krainer tested the newly approved SMA treatment, risdiplam, and another RNA therapeutic, branaplam.
Can AI uncover breast cancer risk factors?
February 26, 2024
This question lies at the heart of a new interdisciplinary collaboration between CSHL’s Camila dos Santos and Peter Koo.
A quiz for the ages
January 29, 2024
Want to know the secret to a long life? So do CSHL scientists. Take this short quiz to see what they’ve found out about aging and longevity.
Joshua-Tor named CSHL Director of Research
January 2, 2024
The Cold Spring Harbor Laboratory professor and HHMI investigator steps into her new role effective January 2, 2024.
Animal behavior quiz
December 20, 2023
Take this short quiz to see how much you know about the science of social behavior.
Smells like learning
October 31, 2023
CSHL research suggests certain neurons help us tell apart different smells while others help us learn to distinguish between similar odors.
You say genome editing, I say natural mutation
October 19, 2023
CSHL scientists have discovered that evolution and genome editing in crops are less predictable than previously thought.
Holy immunity! Bat genes key against COVID, cancer
October 16, 2023
Rapid evolution has streamlined bats’ immune systems. This may explain why they’re resistant to cancer and viruses like Ebola or COVID-19.
The 2023 CSHL Volleyball League Finals
October 11, 2023
With a wide swath of the CSHL community in attendance, we got an up-close view of the action. How close? Think “camera on the ref’s head” close.
Mitra among first awarded NIH BRAIN CONNECTS grant
September 26, 2023
New National Institutes of Health initiative aims to generate an atlas of brain connections, offering new insights into neurological disorders.
Laying the groundwork for drug discoveries
August 8, 2023
A new partnership between CSHL and one of the world’s leading biotech investors could streamline this process and help change society for the better.
Eight serving one: CSHL volleyball mid-season report
August 2, 2023
CSHL’s 32nd Volleyball League season sees eight teams battling for the coveted Tiernan Cup and a year’s worth of bragging rights.
How popular steroids could mess up some cancer treatments
June 23, 2023
Scientists have long wondered how common steroids work and why cancer immunotherapy fails in certain patients. The answers may be one and the same.
The digital dark matter clouding AI
June 5, 2023
Scientists have unknowingly encountered mysterious noise while using AI to decipher our genetic code. CSHL has found a way to cut through the fog.
President’s essay: Bringing bold visions to life
May 26, 2023
CSHL President & CEO Bruce Stillman sees the Laboratory as a global hub for scientific expertise and a powerful launchpad for early-career scientists.
The evolution of autism research
May 25, 2023
The conversation around autism has evolved over the past two decades. So has CSHL research. This retrospective shows how we’ve helped move the needle.
Autism in the family tree
May 23, 2023
CSHL scientists have studied the genetics of autism across hundreds of family trees. This animated video shows what they’ve found.
Siblings with autism share more of dad’s genome, not mom’s
May 22, 2023
CSHL study of more than 6,000 volunteer families overturns a long-held assumption about the genetic origins of autism spectrum disorder.
AI training: A backward cat pic is still a cat pic
May 4, 2023
This basic rule of thumb is helping CSHL’s quantitative biologists train AI to get a better read of the human genome.
How well do you know autism spectrum disorders?
April 24, 2023
April is National Autism Awareness Month. Test your knowledge of autism spectrum disorders with this short quiz.
Isabella Rossellini shares the stage with CSHL
March 28, 2023
Watch as the famed Italian actress chats with neuroscientist Helen Hou about Charles Darwin, women in STEM, stage fright, and much more.
Tour a CSHL lab with film icon Isabella Rossellini
March 3, 2023
This weekend, the worlds of art and science are one at CSHL as Rossellini presents her new play, Darwin’s Smile. Get your sneak peek here.
Follow your nose: Tracking the brain’s smell circuits
February 27, 2023
Take this 8-bit trip along the brain’s olfactory circuits to see what happens up there when you smell something.
Can you outsmart this AI quiz?
February 6, 2023
Think you’re plugged into the latest artificial intelligence advancements? Test your tech knowledge with this quiz on AI and computational biology.
How evolved is your knowledge?
January 26, 2023
Test your knowledge of evolution with this quiz, inspired by the March 2023 performances of Isabella Rossellini’s play, Darwin’s Smile, at CSHL.
Unlocking cancer’s ancestry
December 27, 2022
New software may help reveal the complete connections between ancestry and cancer, which could lead to better, more personalized treatments.
Cold Spring Harbor Laboratory: Foundations for the Future
December 15, 2022
CSHL continues to lead in biomedical sciences by fostering a collaborative, innovative, and high-risk, high-reward research community.
Finding the right AI for you
December 5, 2022
AI’s popularity has reached a point where there are too many options. How do you know which AI is right for you? CSHL scientists have a solution.
Darwin’s Smile explores the cross-section of art and science
December 2, 2022
Isabella Rossellini’s new one-woman show unpacks the origins of emotions, a place where the art of acting and the science of evolution come together.
Welcome to Biology + Beyond
November 14, 2022
CSHL President and CEO Bruce Stillman introduces a special issue of Nautilus magazine now online, featuring the Lab’s latest groundbreaking research
Mapping the path from smell to perception
October 27, 2022
Smell remains the most mysterious of our five senses, but CSHL neuroscientists are now closer than ever to understanding it.
Even fruit flies count
October 25, 2022
Fruit flies know if they’ve smelled an odor once, twice, many times, or never before.
CSHL high schoolers finish top 10 in 2022 DREAM Challenge
October 7, 2022
The high school team competed against universities and private labs to build a computer program for predicting gene expression in yeast.
Benjamin Cowley joins CSHL neuroscience faculty
September 27, 2022
The Cowley group creates computer models to study how the brain processes information gathered by the senses.
Exposing the evolutionary weak spots of the human genome
September 22, 2022
Researchers built a computer program that tracks harmful mutations throughout human evolution. It may help uncover the origins of genetic diseases.
How the thymus trains T cells to fight infections
August 2, 2022
CSHL scientists identified, for the first time, the RNA in humans used to train T cells to attack dangerous or foreign proteins in the body.
CSHL welcomes neuroscientist Helen Hou
July 1, 2022
The Hou Lab will explore how the brain controls movement and behavior, including how it makes facial expressions and conveys emotion.
Building better AI with the power of neuroscience
June 8, 2022
Artificial Intelligence (AI) experts at CSHL are creating better AI by deciphering brain circuits.
President’s essay: Foundations for the future
May 25, 2022
Strategically designed to spark scientific exchange and inspiration, CSHL is a unique research and education environment for advancing science.
New CSHL website brings together sorghum researchers
May 9, 2022
Plant researchers and breeders are now using a website created by CSHL to get the latest intel on sorghum crop research.
The race to protect sweet corn
April 22, 2022
Breeding a variety that can withstand disease and taste better too
Do you have the dirt on plant research?
March 31, 2022
New research is constantly sprouting. Take this quiz and test your plant knowledge.
Regeneron competition honors CSHL high school researchers
March 22, 2022
Three high school student researchers at CSHL were among Regeneron Science Talent Search’s top 300 scholars. One made it to the final competition.
Deciphering algorithms used by ants and the Internet
March 1, 2022
Researchers discovered the same optimization algorithm used by Internet engineers is used by ants when they forage for food.
AI is helping scientists explain our brain
February 28, 2022
Neuroscientists are turning to artificial intelligence to help them understand the brain, but what if AI misses the true story?
Getting a step ahead of TB’s drug resistance evolution
February 15, 2022
Mutations are not random, with some kinds of changes occurring more often than others. CSHL researchers may be able to predict which direction evolution is li
Brain waves churn differently when paying attention
February 2, 2022
See the shapes and speeds of electrical waves in the brain change in response to attentiveness.
Finding structure in the brain’s static
February 1, 2022
CSHL researchers found that the brain’s state of attentiveness may be encoded in the shapes and speeds of slow electrical waves.
CSHL Fellow Hannah Meyer wins UK Biobank researcher award
November 17, 2021
The UK Biobank recognized CSHL Fellow Hannah Meyer’s scientific achievements in understanding the inner workings of the human heart.
The rise of RNA therapeutics
October 14, 2021
RNA has been making waves as a new approach to prevent or treat diseases, including COVID-19 and spinal muscular atrophy.
Building on 150 years of neuroanatomy
October 7, 2021
Learn more about how researchers reached a milestone in a years-long effort to catalog the cells of the human, mouse, and monkey brains.
Think a census of humans is hard? Try counting their brain cells!
October 6, 2021
CSHL researchers and other collaborators reached a milestone in a years-long effort to catalog the cells of the human, mouse, and monkey brains.
Calculating the path of cancer
October 4, 2021
A new mathematical approach is helping cancer researchers at CSHL determine how mutations lead to different behaviors in cancerous cells.
Team P.E. Revival wins CSHL volleyball championship
September 29, 2021
Team PE Revival won the thirtieth annual CSHL beach volleyball tournament by winning two out of three matches.
CSHL Ph.D. program: Graduating class of 2021
August 22, 2021
The CSHL School of Biological Sciences awarded Ph.D. degrees to seven students this year, who describe some of their experiences.
The secret history of corn is revealed in its genome
August 5, 2021
For the first time, scientists have assembled in-depth maps of dozens of corn genomes, filling in gaps related to key agricultural traits.
URP: Summer camp for undergrads
July 29, 2021
The Undergraduate Research Program brings college students from around the world to CSHL for a summer of research and fun.
How to outwit evolution
July 21, 2021
CSHL Assistant Professor David McCandlish uses statistical methods to predict the evolution of antibiotic resistance in bacteria.
Using “guilt by association” to classify cells
July 14, 2021
Using a new computational statistics tool, CSHL researchers classify cells to understand how an organism functions.
Solving genetic disease puzzles with quantitative biology
June 17, 2021
CSHL quantitative biologist Jesse Gillis teams up with an immunology specialist at Northwell Health to analyze a complex genetic disorder.
Research matters
June 8, 2021
Innovative research and educational activities never stopped during the COVID-19 pandemic.
Let’s talk about the elephant in the data
June 3, 2021
How much prior knowledge does a machine learning computer need to find the truth? CSHL Professor Partha Mitra looks to human brains for an answer.
Making AI algorithms show their work
May 13, 2021
AI machines are often better than humans at discerning patterns. CSHL researchers developed a way to find out why.
How a bad day at work led to better COVID predictions
May 3, 2021
A CSHL computer scientist and an MSK infectious disease physician developed a method for predicting COVID-19 severity in cancer patients.
Are you sure you heard that sound?
April 14, 2021
CSHL and Washington University of St. Louis researchers studied hallucination-like perceptions in humans and mice that mimic schizophrenia symptoms.
Local Regeneron scholars celebrated by Congressman Suozzi
March 5, 2021
Over twenty local high school students were named scholars in the Regeneron Science Talent Search national competition and awarded $2,000 scholarships.
In addition to its research activities, the SCQB serves as a hub for education, training and research in the quantitative life sciences.
Events
QB/AI Seminar Series
The QB/AI Seminar Series is a weekly symposium featuring a rotating roster of graduate students, postdocs and invited guests. Seminars are held most Wednesdays at noon during the academic calendar year.
QB Meetings and Conferences
Members and Associate Members of the SCQB faculty organize relevant QB Meetings and Conferences hosted at CSHL and around the NY area.
- Probabilistic Modeling in Genomics
- Biological Data Science
- NY Populations Genomics Workshop
QB Scientific Tea
The SCQB community which includes faculty, postdocs, graduate students, staff and special guests are invited to attend weekly catered informal gatherings to discuss their research and other relevant topics.
Journal Clubs
Members of the SCQB host a bi-weekly Sequence/Function Journal club and a monthly Deep Learning journal club during the academic calendar year.
Opportunities for Postdoctoral Researchers
The CSHL Fellows Program
The CSHL Fellows Program supports research fellows, who function independently but with mentoring from the senior faculty. The program is designed for exceptional quantitative biologists who have recently received their Ph.D. or M.D. degree and who are sufficiently talented and experienced to forgo standard postdoctoral training.
Interdisciplinary Scholars in Experimental and Quantitative Biology Program (ISEQB)
The Interdisciplinary Scholars in Experimental and Quantitative Biology (ISEQB) is an innovative funding opportunity for postdoctoral research open to applications in all areas of research at CSHL, including genetics, cancer, plant biology and neuroscience. The ISEQB is designed to help recruit new postdocs or fund existing CSHL postdocs who are interested in both wet-lab and dry-lab research. This program aims to catalyze collaborative research as well as promote the growth of the QB community at CSHL.
Course Work
School of Biological Sciences QB Bootcamp at CSHL
The School of Biological Sciences QB Bootcamp is a 2.5-day rapid introduction to Python and the computer cluster at CSHL taught each Fall by the SCQB faculty to provide incoming students with working knowledge in programming in preparation for the full-semester Specialized Discipline Course in Quantitative Biology.
Specialized Discipline Course in Quantitative Biology at CSHL
The Specialized Discipline Course in Quantitative Biology is a 16-week course that aims to equip incoming students with basic training in computer programming, modern statistical methods and physical biology. Using a probabilistic and Bayesian approach, the course covers probabilities, statistical fluctuations, Bayesian inference, significance testing, fluctuations, diffusion, information theory, neural signal processing, dimensional reduction, Monte Carlo methods, population genetics and DNA sequence analyses.
Advanced Coursework in Quantitative Biology
The Simons Center for Quantitative Biology (SCQB) provides Advanced Coursework in Quantitative Biology to graduate students, postdocs and scientific staff through independent study programs and online coursework.
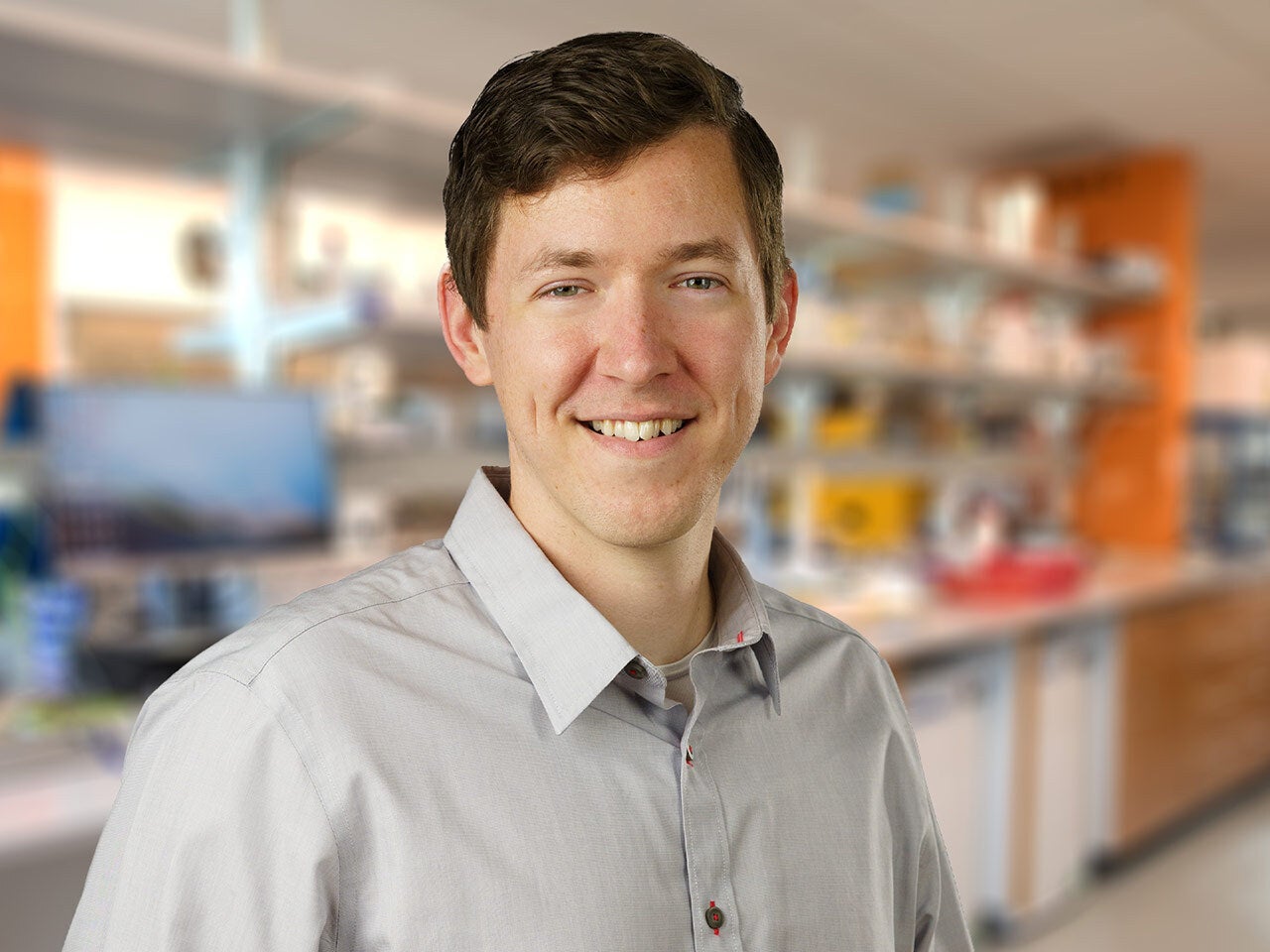
Benjamin Cowley
How do we identify and describe the step-by-step computations of the brain? The Cowley group identifies data-driven models of neural responses and behavior by coupling data collection with model training during closed-loop experiments. We condense these models into compact, interpretable forms—allowing us to describe the complicated computations of the brain in a clear and concise way.
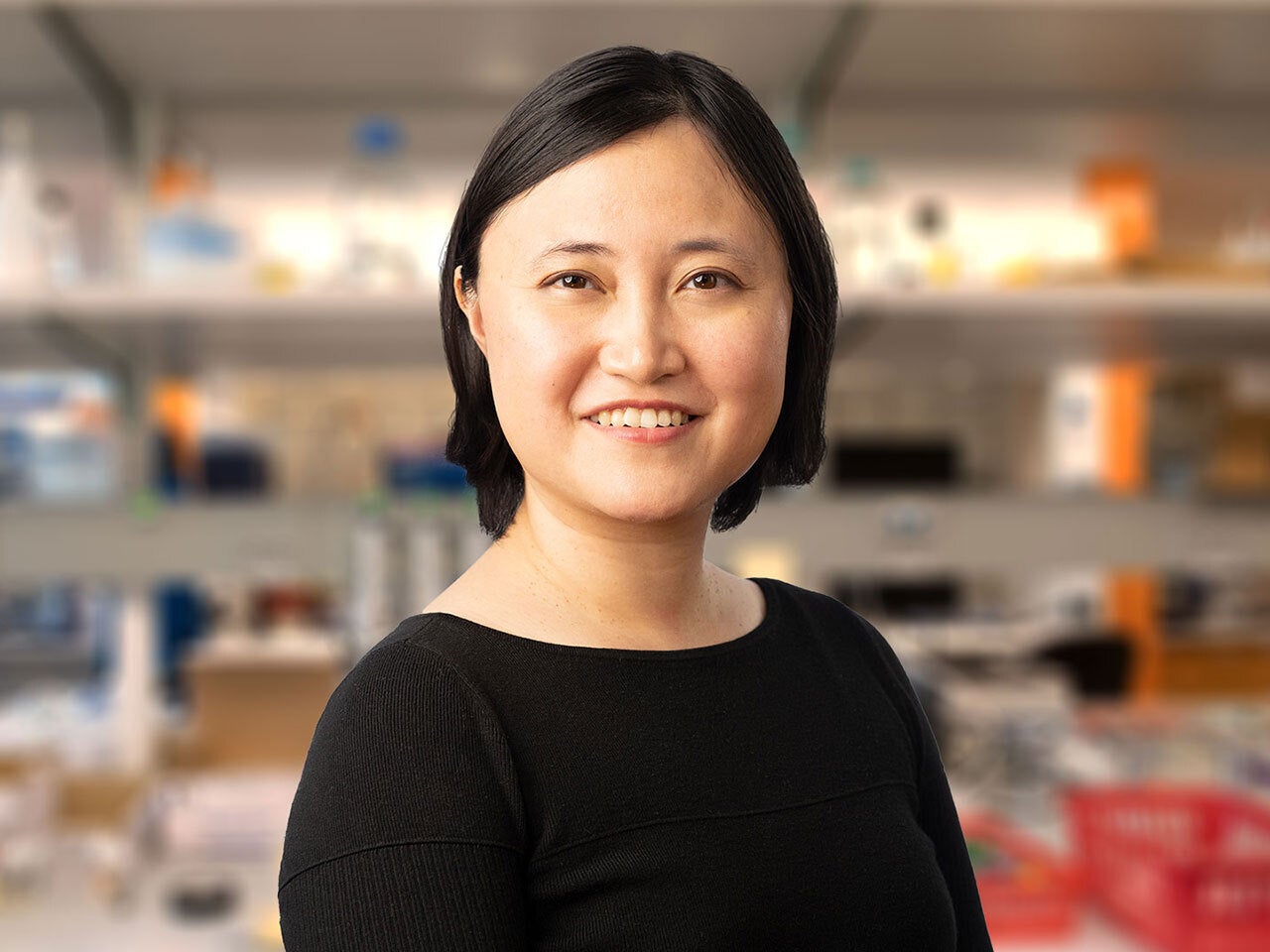
Helen Hou
The brain-body interaction is a two-sided coin: The brain can control movement of the body to fulfill behaviors, and behavior itself can affect brain function. We study how the brain orchestrates motor and physiological control in natural and innate behaviors, focusing on facial expression.
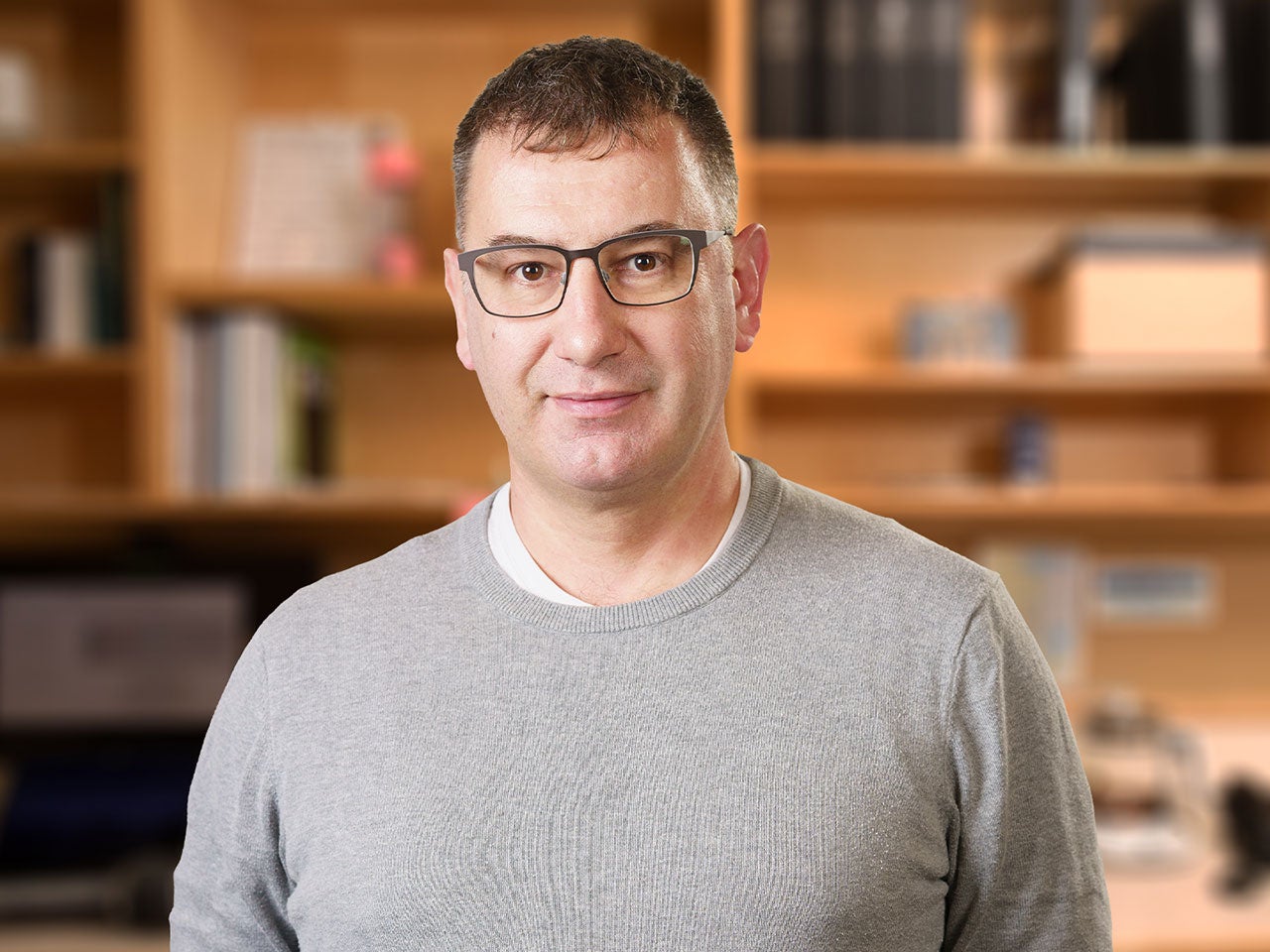
Ivan Iossifov
Every gene has a job to do, but genes rarely act alone. Biologists have built models of molecular interaction networks that represent the complex relationships between thousands of different genes. I am using computational approaches to help define these relationships, work that is helping us to understand the causes of common diseases including autism, bipolar disorder, and cancer.
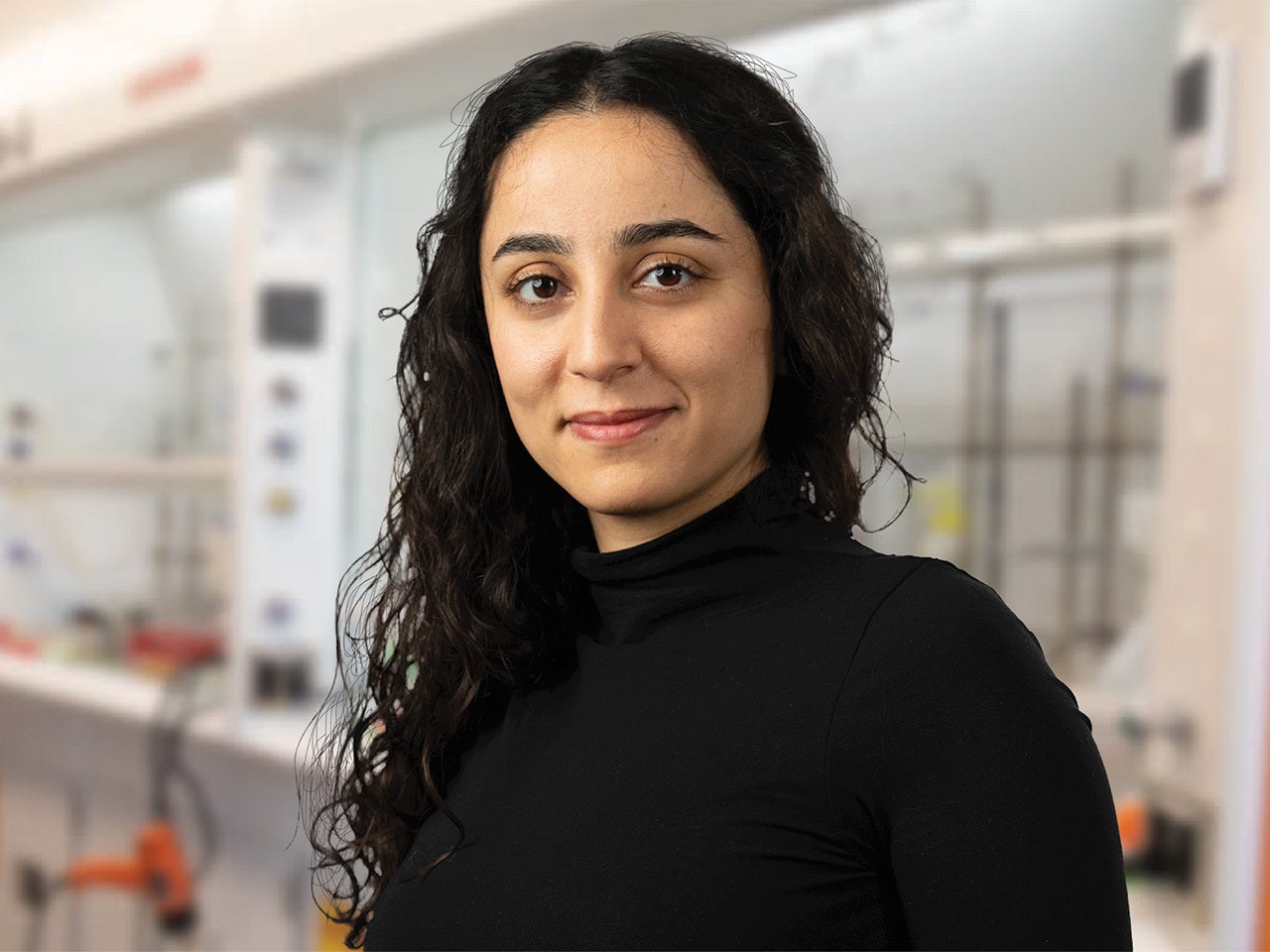
Mitra Javadzadeh
Sensory stimuli evoke activity in millions of neurons spread across multiple brain regions. This activity evolves over time, not only due to the constantly changing outside world, but also as a result of the internal interactions within these brain-wide networks. We aim to understand how distributed neural population dynamics are organized, and how they underlie our robust and yet flexible perception.
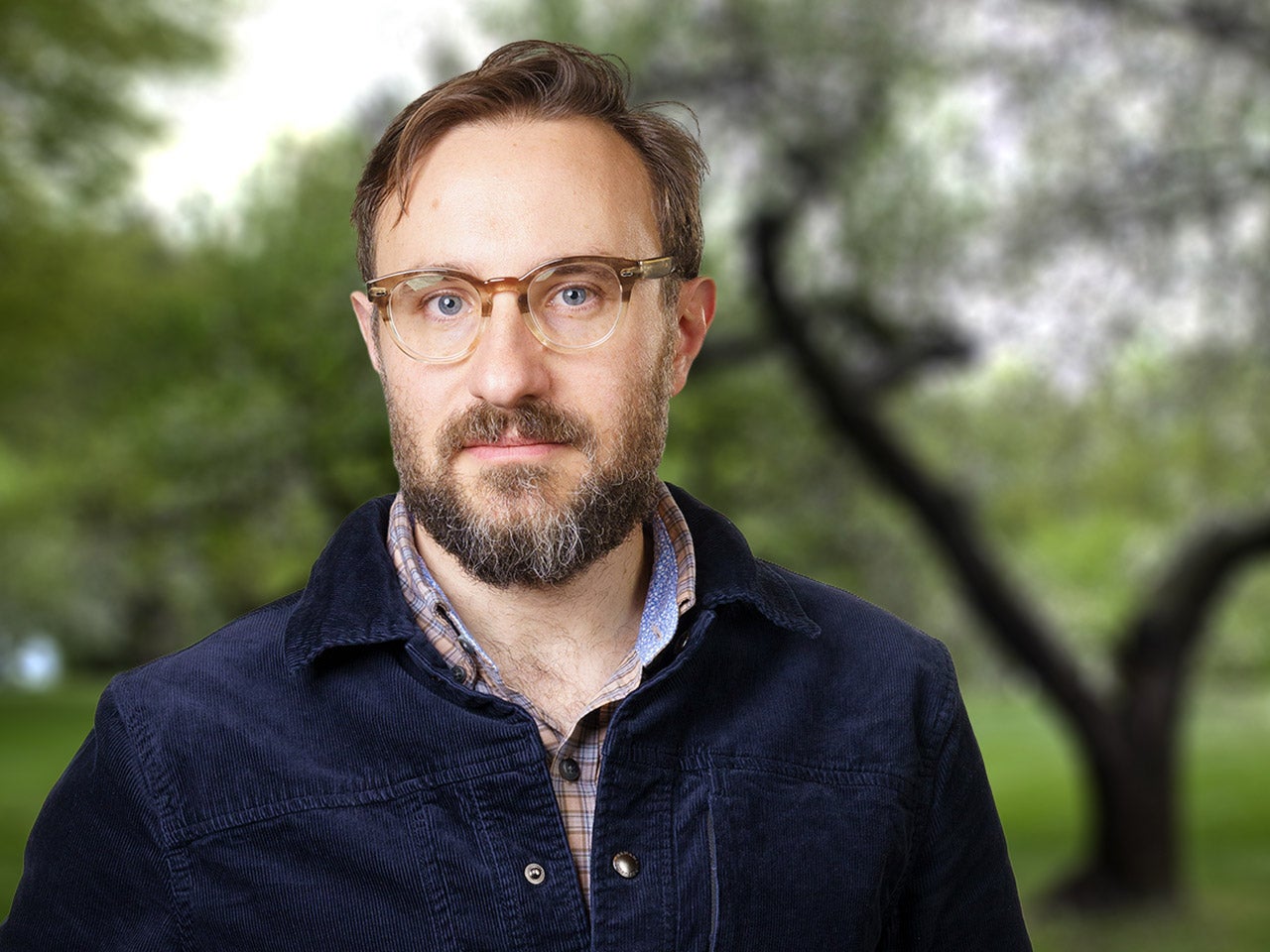
Justin Kinney
Research in the Kinney Lab combines mathematical theory, machine learning, and experiments in an effort to illuminate how cells control their genes. These efforts are advancing the fundamental understanding of biology and biophysics, as well as accelerating the discovery of new treatments for cancer and other diseases.
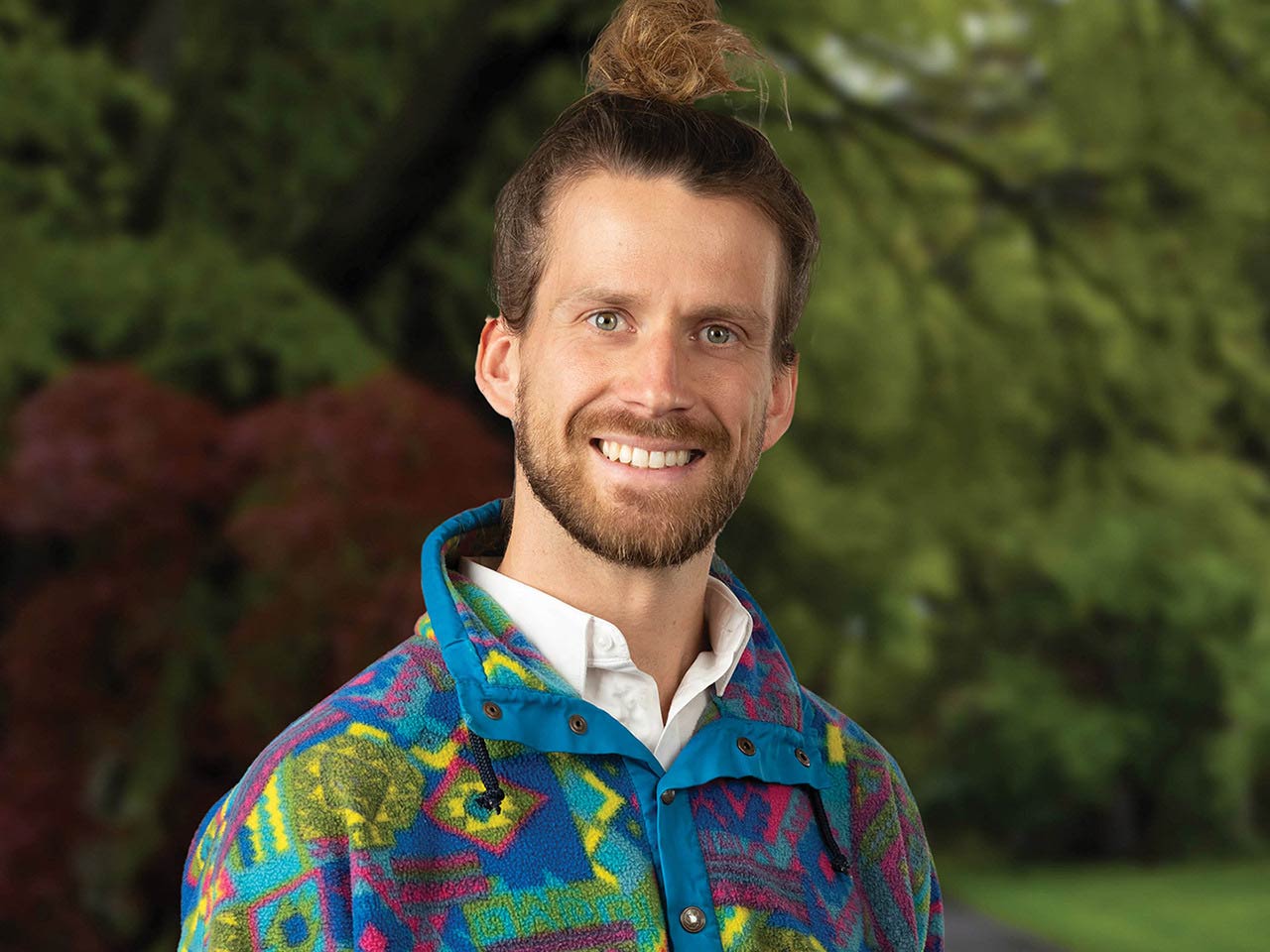
David Klindt
Our research explores how biological systems, such as the brain, learn from sensory data and generalize knowledge to new situations, inspiring the development of more robust artificial intelligence models. By investigating neural representations and leveraging expertise in computational neuroscience and AI, we aim to uncover groundbreaking insights at the intersection of biology and technology.
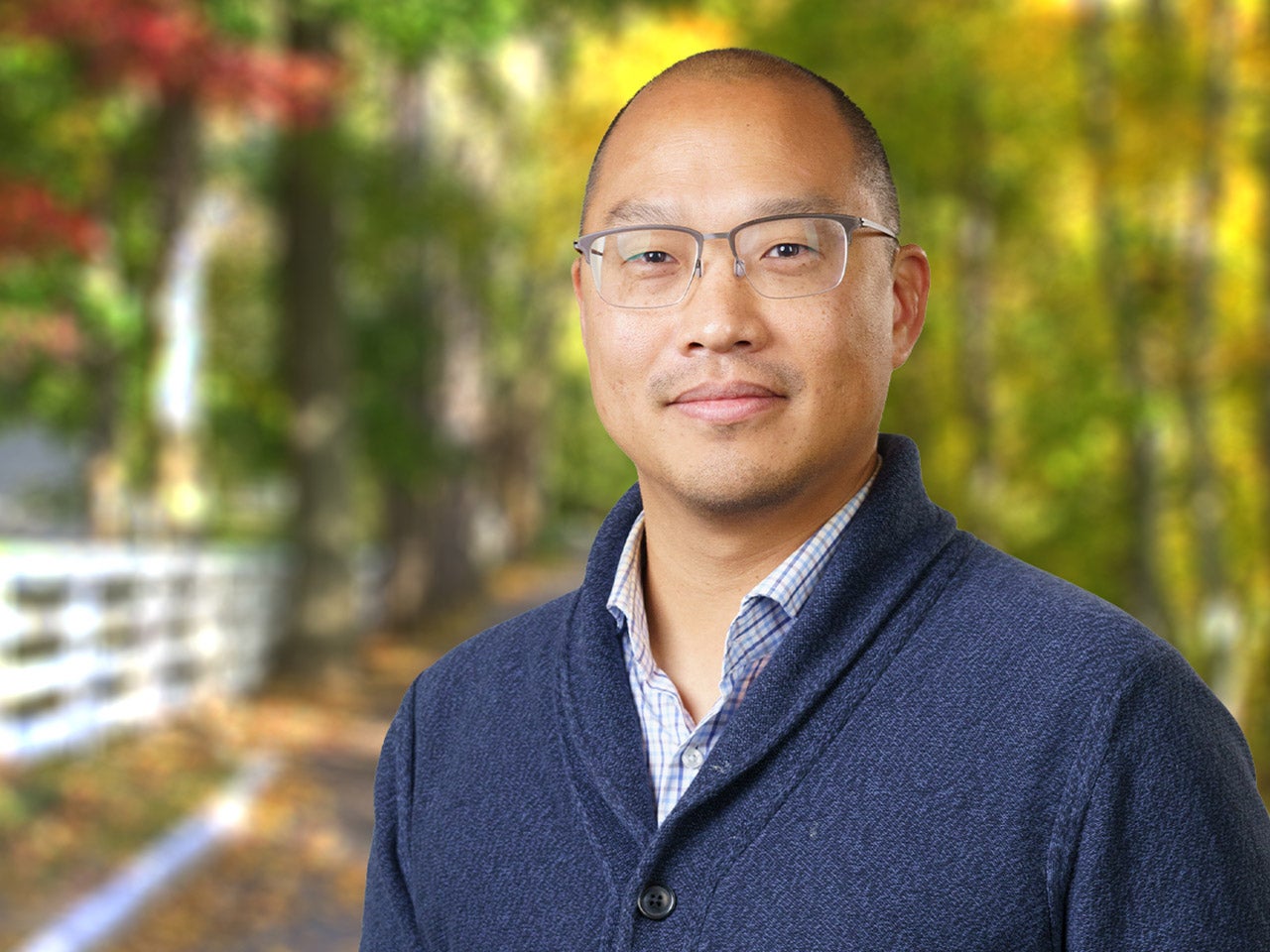
Peter Koo
Deep learning has the potential to make a significant impact in basic biology and cancer, but a major challenge is understanding the reasons behind their predictions. My research develops methods to interpret this powerful class of black box models, with a goal of elucidating data-driven insights into the underlying mechanisms of sequence-function relationships.
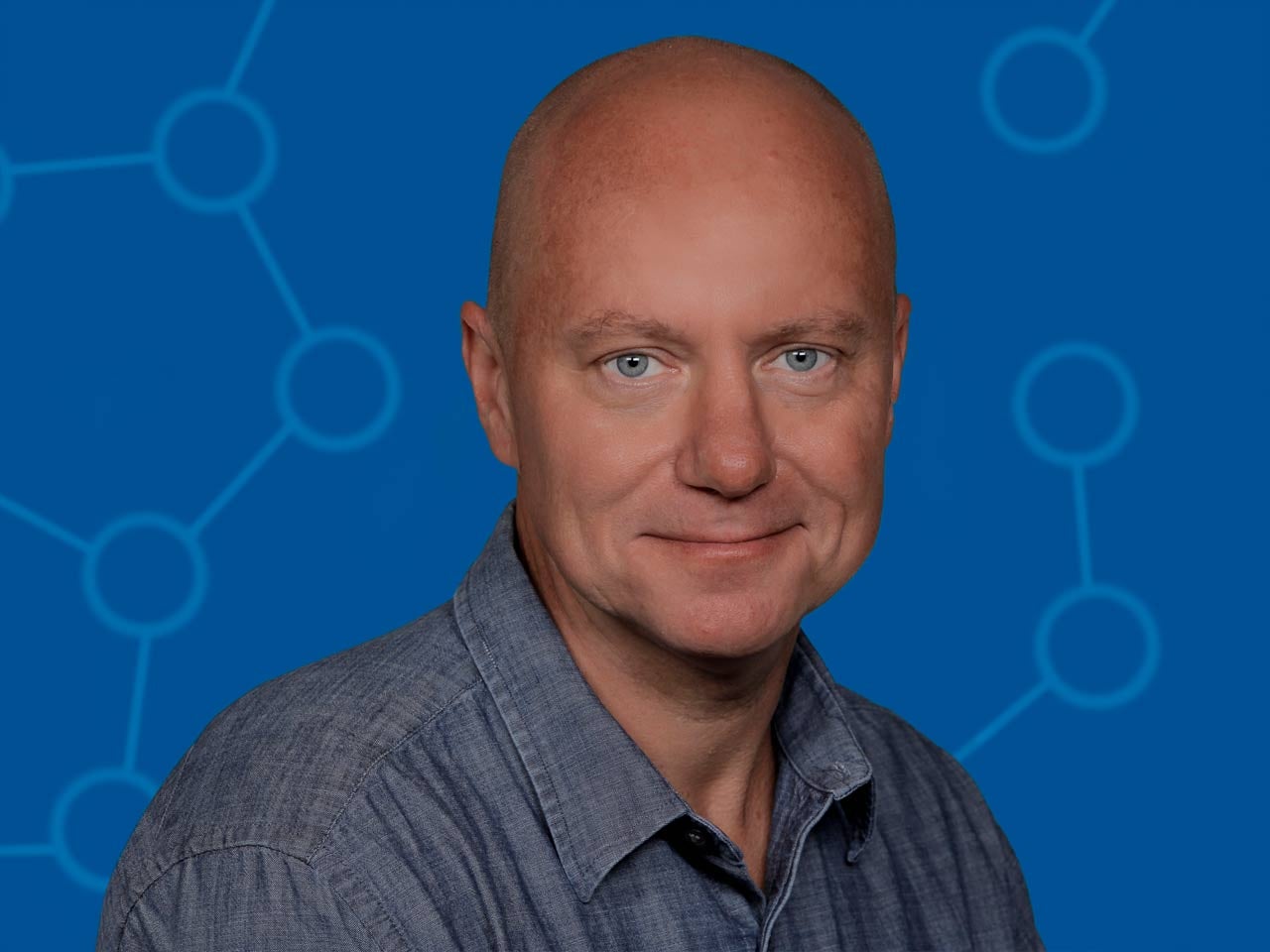
Alexei Koulakov
The complexity of the mammalian brain challenges our ability to explain it. My group applies methods from mathematics and theoretical physics to understand the brain. We are generating novel ideas about neural computation and brain development, including how neurons process information, how brain networks assemble during development, and how brain architecture evolved to facilitate its function.
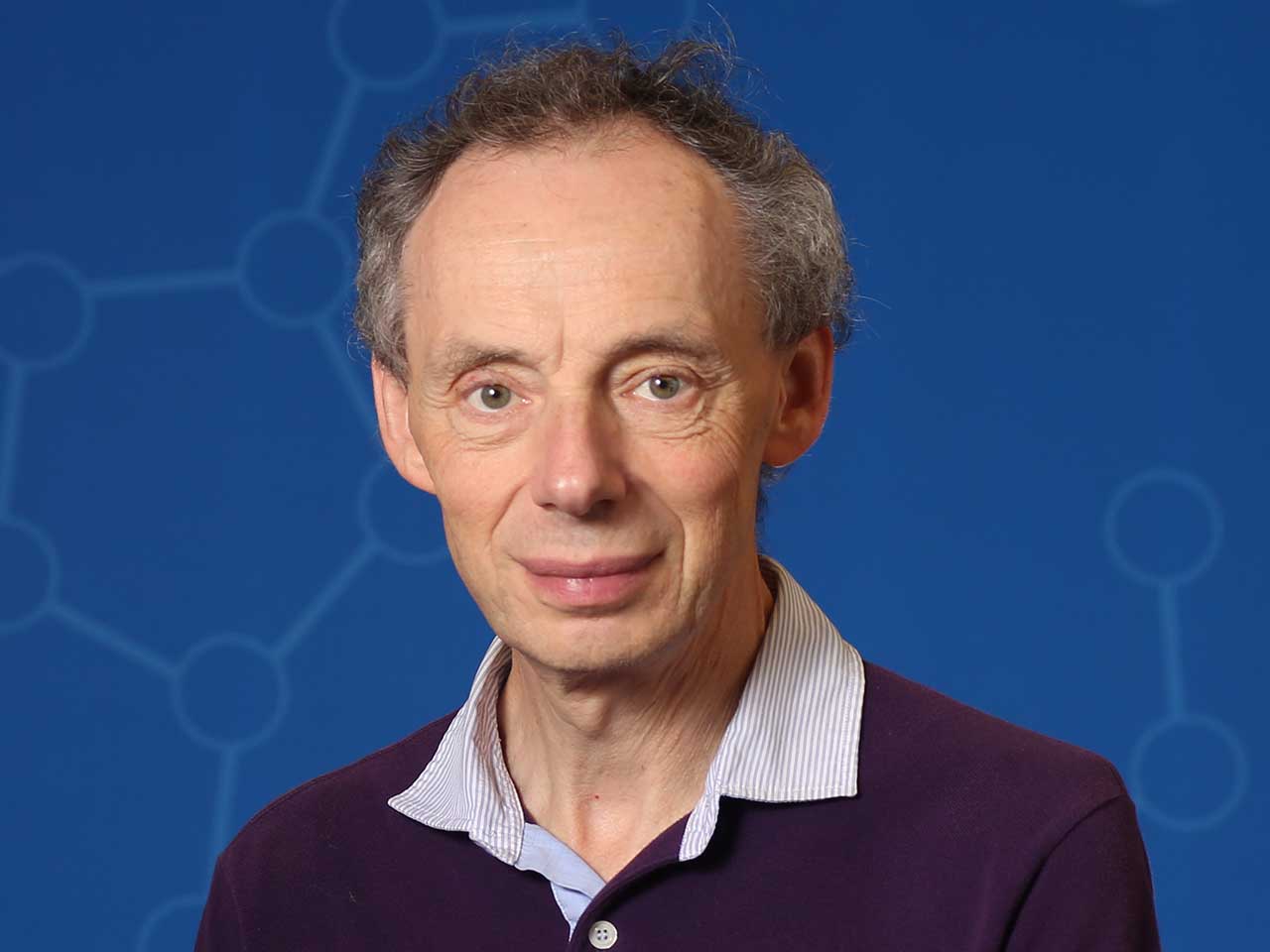
Alexander Krasnitz
Many types of cancer display bewildering intra-tumor heterogeneity on a cellular and molecular level, with aggressive malignant cell populations found alongside normal tissue and infiltrating immune cells. I am developing mathematical and statistical tools to disentangle tumor cell population structure, enabling an earlier and more accurate diagnosis of the disease and better-informed clinical decisions.
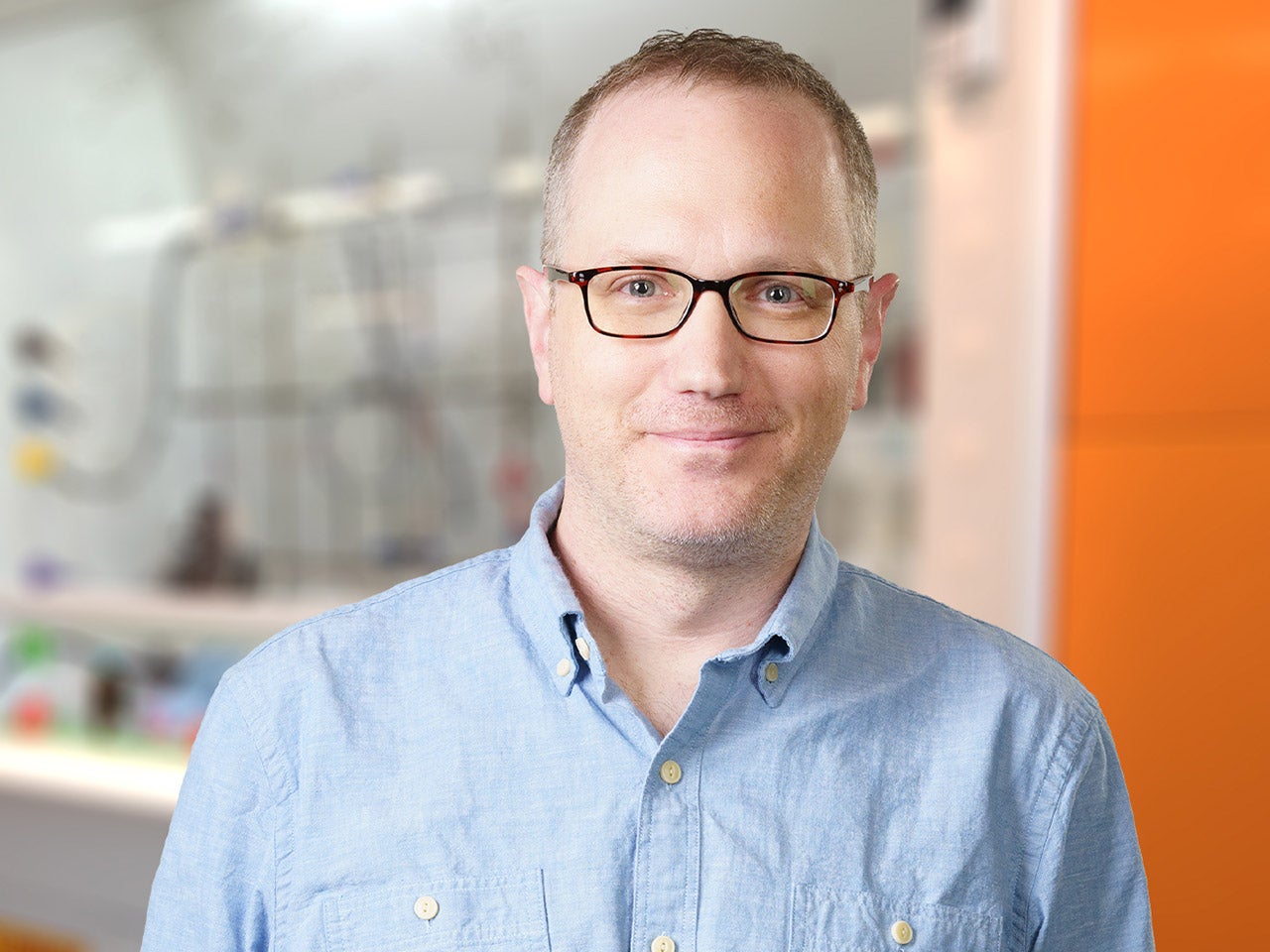
Dan Levy
We have recently come to appreciate that many unrelated diseases, such as autism, congenital heart disease and cancer, are derived from rare and unique mutations, many of which are not inherited but instead occur spontaneously. I am generating algorithms to analyze massive datasets comprising thousands of affected families to identify disease-causing mutations.
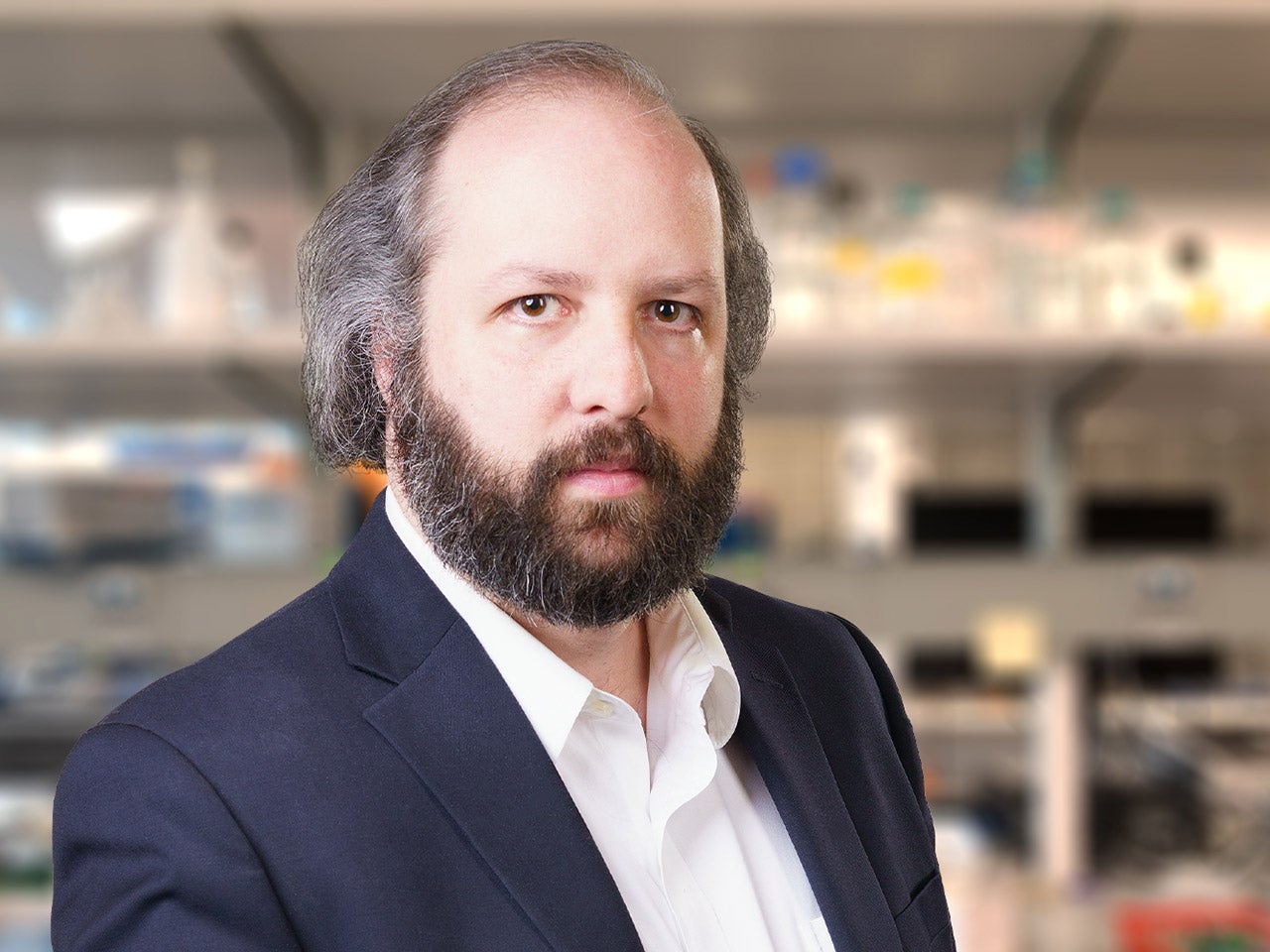
David McCandlish
Some mutations are harmful but others are benign. How can we predict the effects of mutations, both singly and in combination? Using data from experiments that simultaneously measure the effects of thousands of mutations, I develop computational tools to predict the functional impact of mutations and apply these tools to problems in protein design, molecular evolution, and cancer.
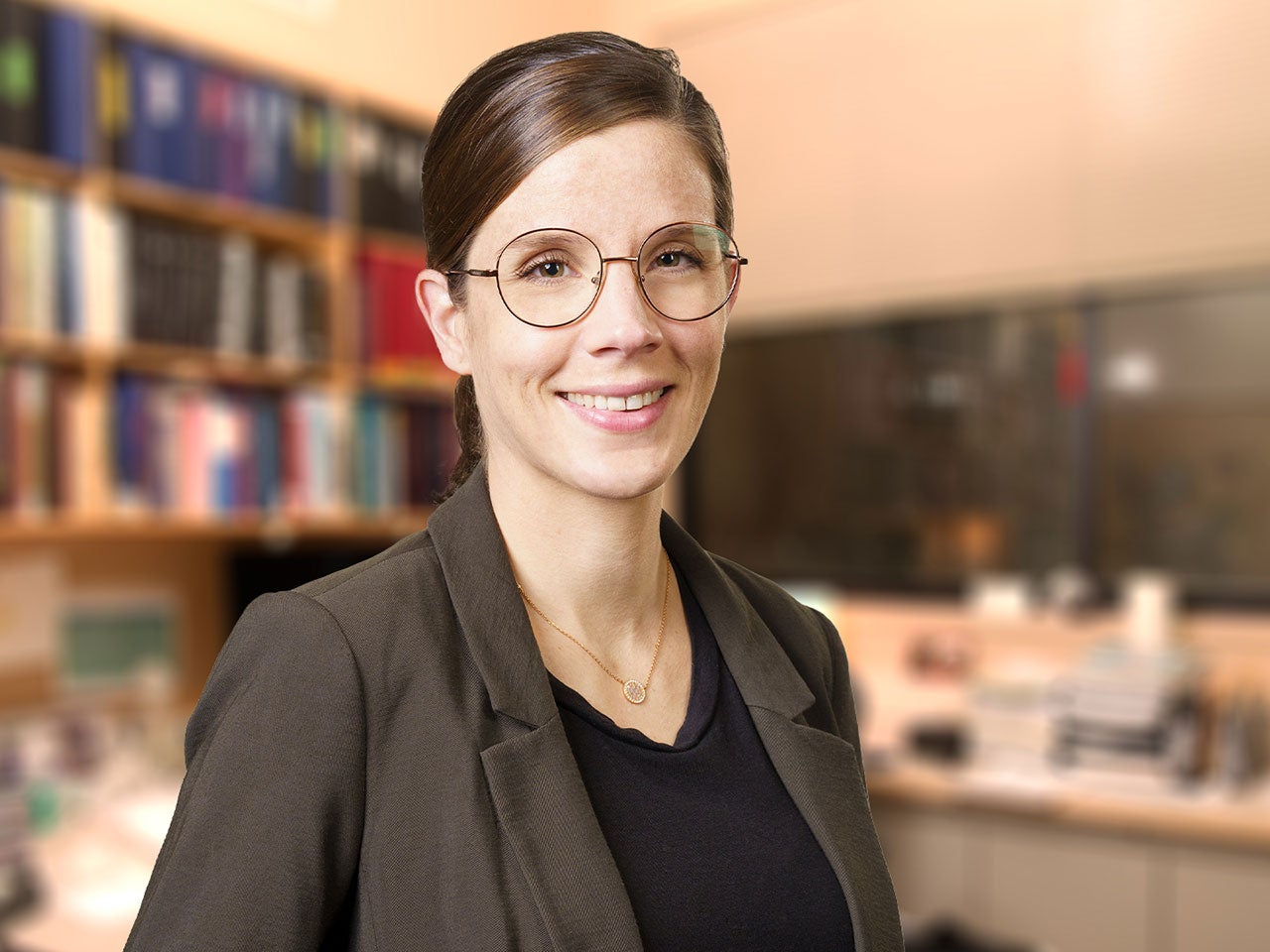
Hannah Meyer
A properly functioning immune system must be able to recognize diseased cells and foreign invaders among the multitude of healthy cells in the body. This ability is essential to both prevent autoimmune diseases and fight infections and cancer. We study how a specific type of immune cells, known as T cells, are educated to make this distinction during development.
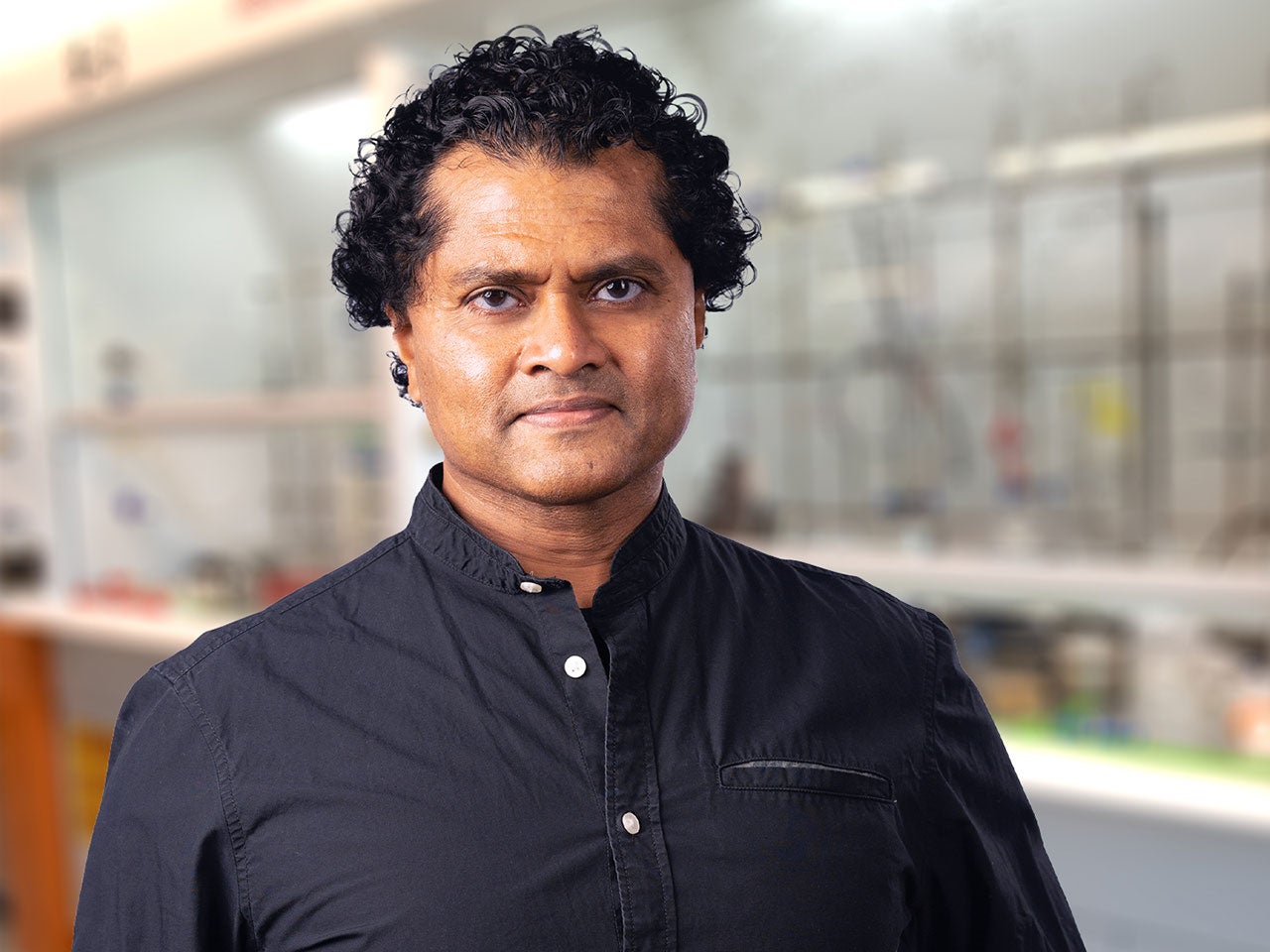
Partha Mitra
A theoretical physicist by training, my research is centered around intelligent machines. I do both theoretical and experimental work. The theoretical work is focussed on analyzing distributed/networked algorithms in the context of control theory and machine learning, using tools from statistical physics. My lab is involved in brain-wide mesoscale circuit mapping in the Mouse as well as in the Marmoset. An organizing idea behind my research is that there may be common underlying mathematical principles that constrain evolved biological systems and human-engineered systems.
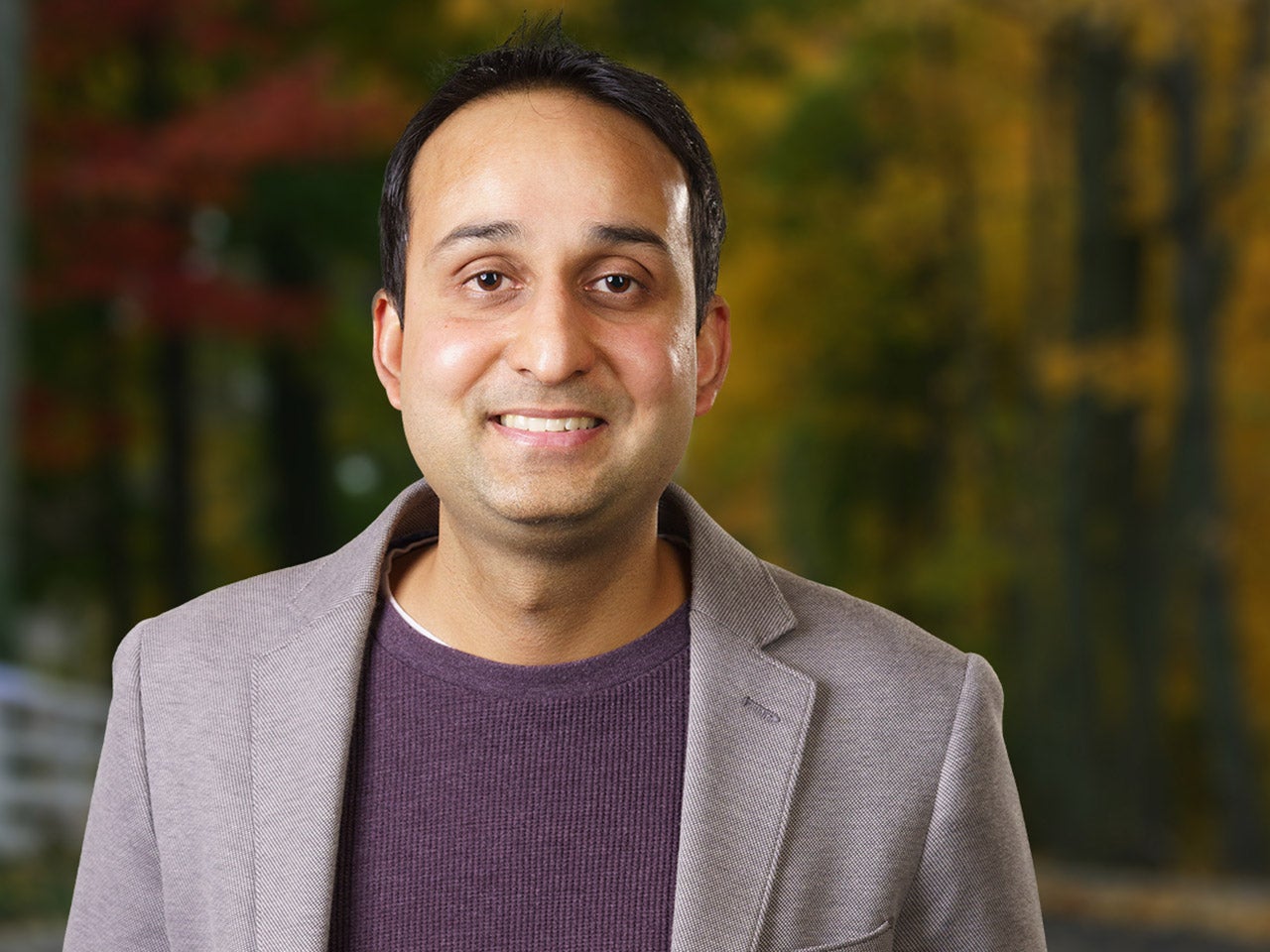
Saket Navlakha
Biological systems must solve problems to survive, and their solutions can be viewed as “algorithms.” Our goal is to uncover these algorithms, translate them to improve computer science, and use them to spark new hypotheses about biological function and dysfunction.
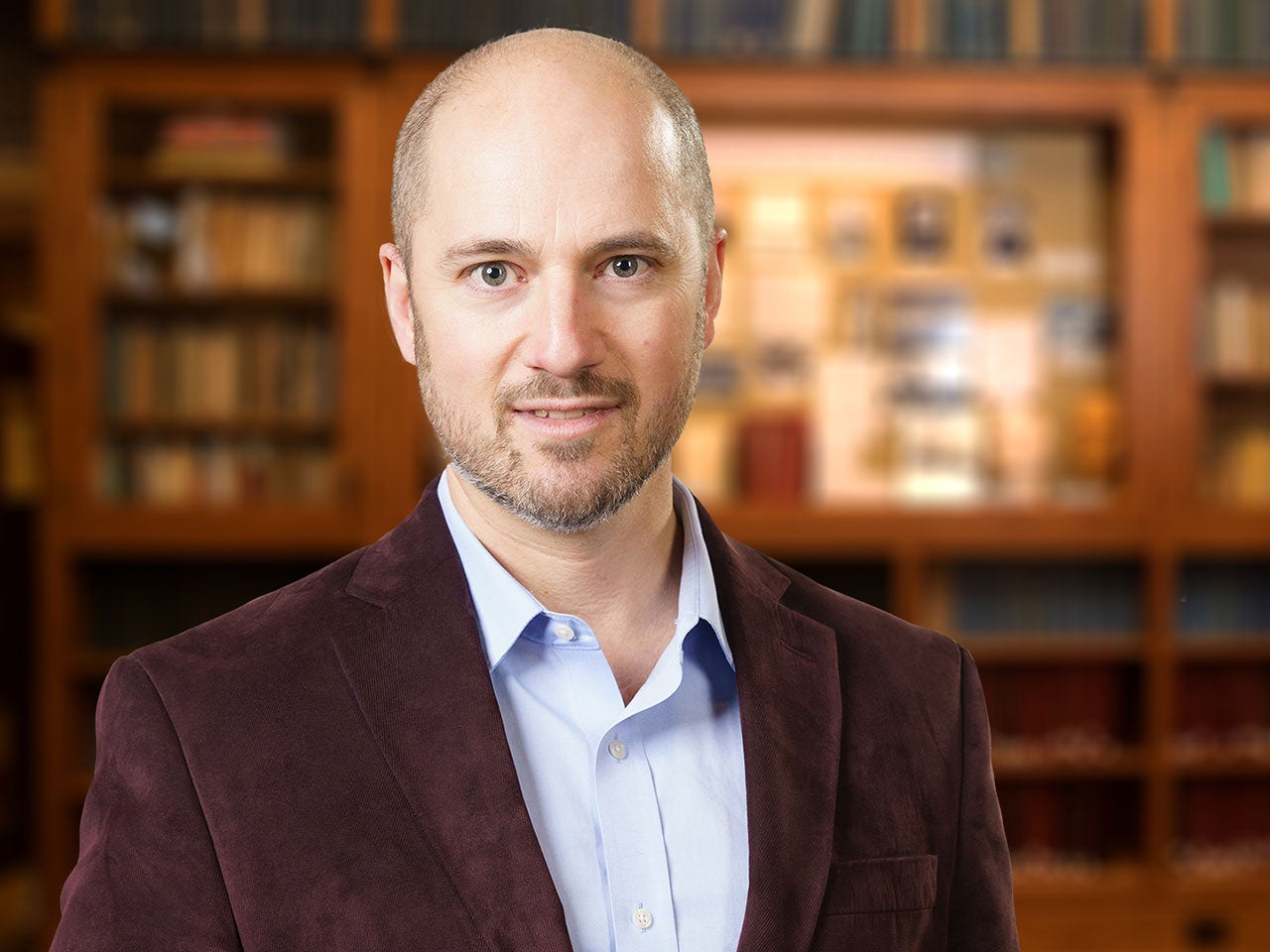
Adam Siepel
I am a computer scientist who is fascinated by the challenge of making sense of vast quantities of genetic data. My research group focuses in particular on questions involving molecular evolution and transcriptional regulation, with applications to cancer and other diseases as well as to plant breeding and agriculture.
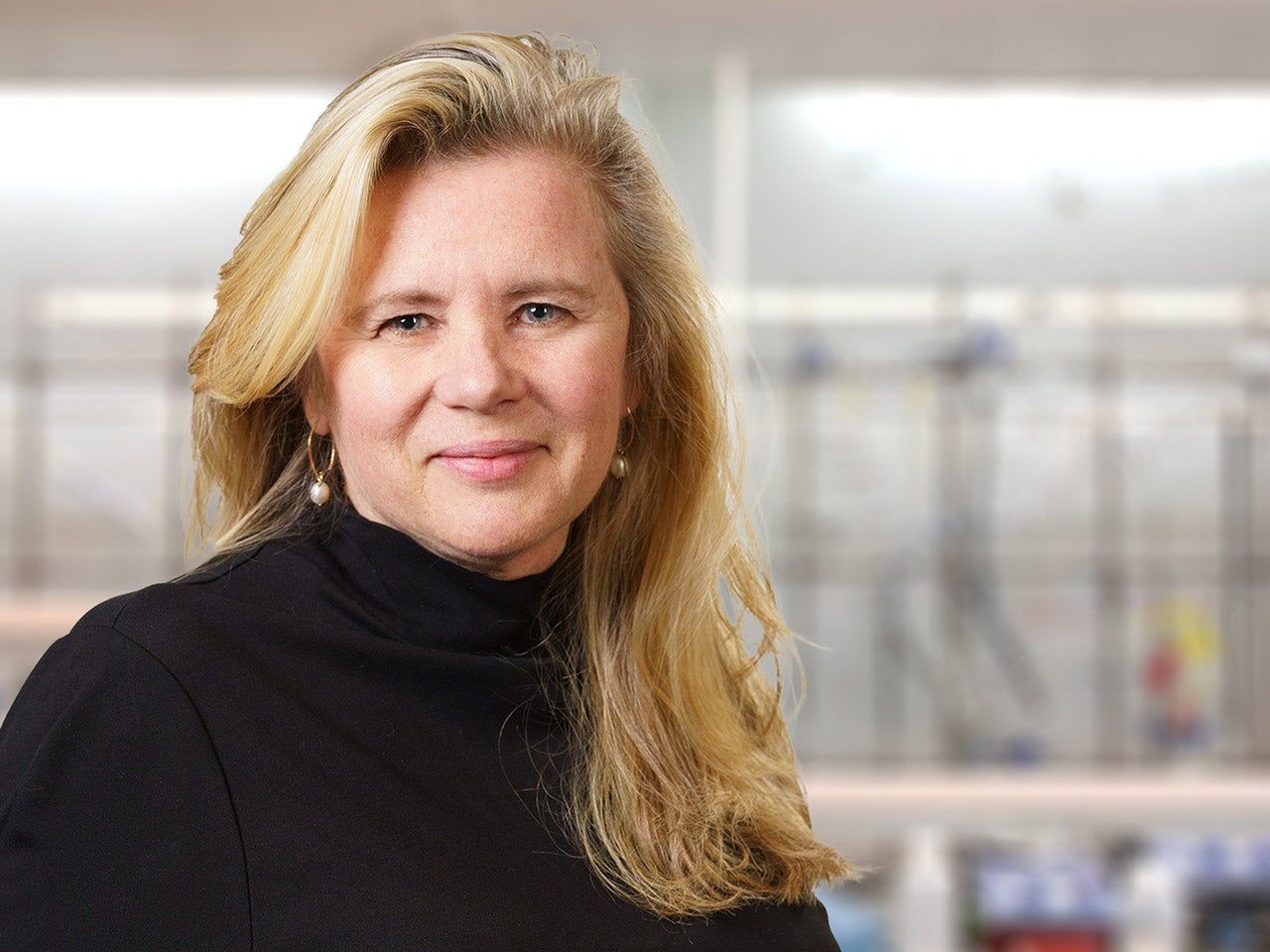
Doreen Ware
When we think of evolution, we often think about physical changes, like a plant developing broader leaves to collect more solar energy. Such evolution actually occurs within the plant’s DNA. I am using computational analysis and modeling to visualize how plant genomes have evolved over time, particularly those of staple crops. We are learning from this work to improve the range and yield of modern plants.