The human genome is three billion letters of code, and each person has millions of variations. While no human can realistically sift through all that code, computers can. Artificial intelligence (AI) programs can find patterns in the genome related to disease much faster than humans can. They also spot things that humans miss. Someday, AI-powered genome readers may even be able to predict the incidence of diseases from cancer to the common cold. Unfortunately, AI’s recent popularity surge has led to a bottleneck in innovation.
“It’s like the Wild West right now. Everyone’s just doing whatever the hell they want,” says Cold Spring Harbor Laboratory (CSHL) Assistant Professor Peter Koo. Just like Frankenstein’s monster was a mix of different parts, AI researchers are constantly building new algorithms from various sources. And it’s difficult to judge whether their creations will be good or bad. After all, how can scientists judge “good” and “bad” when dealing with computations that are beyond human capabilities?
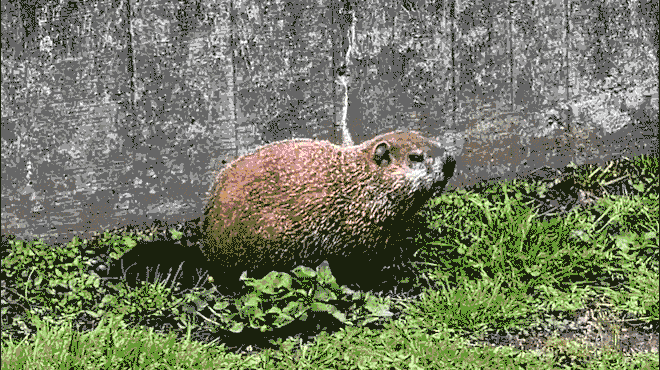
That’s where GOPHER, the Koo lab’s newest invention, comes in. GOPHER (short for GenOmic Profile-model compreHensive EvaluatoR) is a new method that helps researchers identify the most efficient AI programs to analyze the genome. “We created a framework where you can compare the algorithms more systematically,” explains Ziqi Tang, a graduate student in Koo’s laboratory.
GOPHER judges AI programs on several criteria: how well they learn the biology of our genome, how accurately they predict important patterns and features, their ability to handle background noise, and how interpretable their decisions are. “AI are these powerful algorithms that are solving questions for us,” says Tang. But, she notes:
“One of the major issues with them is that we don’t know how they came up with these answers.”
GOPHER helped Koo and his team dig up the parts of AI algorithms that drive reliability, performance, and accuracy. The findings help define the key building blocks for constructing the most efficient AI algorithms going forward. “We hope this will help people in the future who are new to the field,” says Shushan Toneyan, another graduate student at the Koo lab.
Imagine feeling unwell and being able to determine exactly what’s wrong at the push of a button. AI could someday turn this science-fiction trope into a feature of every doctor’s office. Similar to video-streaming algorithms that learn users’ preferences based on their viewing history, AI programs may identify unique features of our genome that lead to individualized medicine and treatments. The Koo team hopes GOPHER will help optimize such AI algorithms so that we can trust they’re learning the right things for the right reasons. Toneyan says:
“If the algorithm is making predictions for the wrong reasons, they’re not going to be helpful.”
Written by: Luis Sandoval, Communications Specialist | sandova@cshl.edu | 516-367-6826
Funding
Simons Center for Quantitative Biology at Cold Spring Harbor Laboratory, National Institutes of Health
Citation
Toneyan, S., Tang, Z., et al., “Evaluating deep learning for predicting epigenomic profiles”, Nature Machine Intelligence, December 5, 2022. DOI: 10.1038/s42256-022-00570-9
Principal Investigator
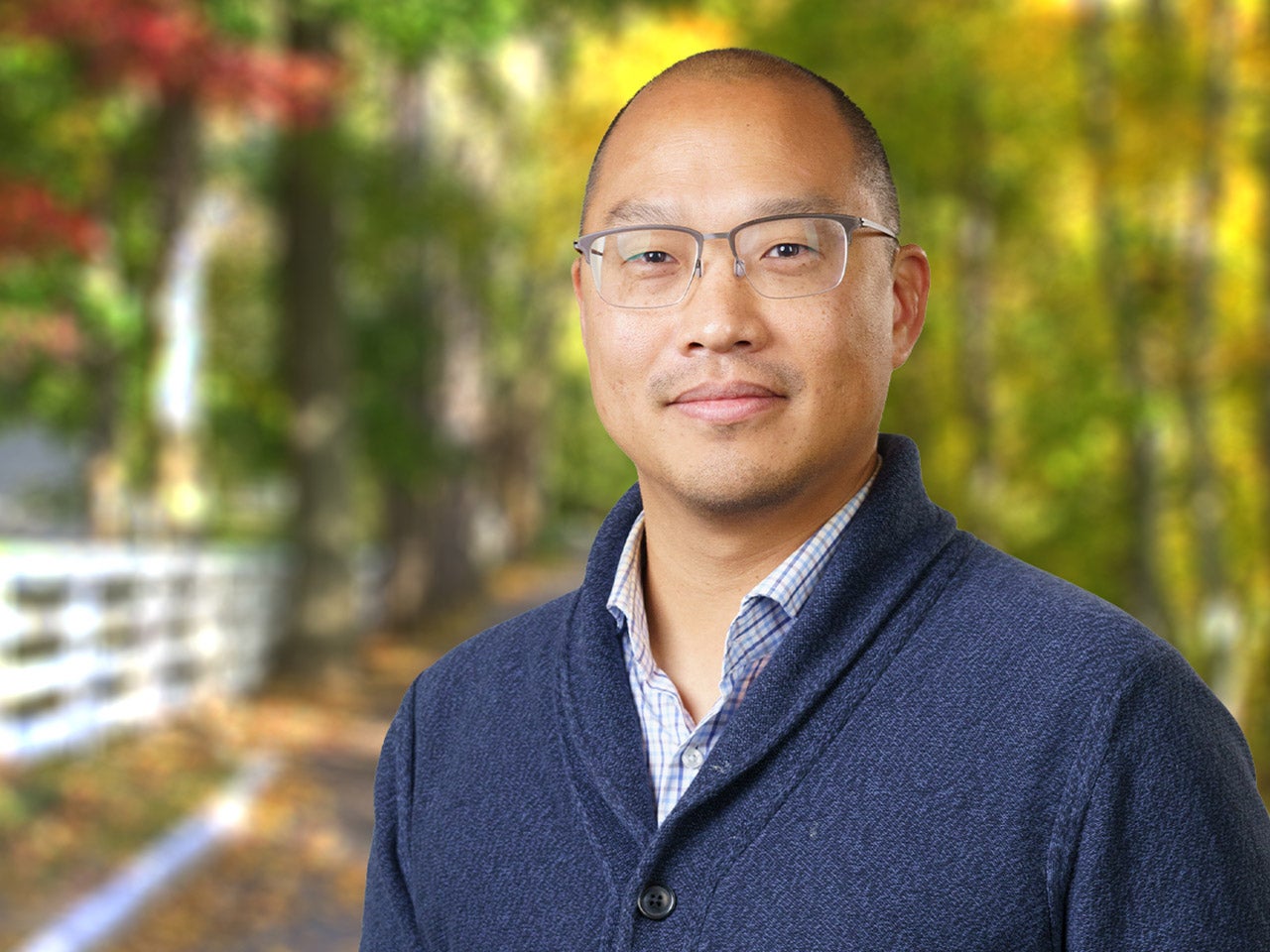
Peter Koo
Associate Professor
Cancer Center Member
Ph.D., Yale University, 2015