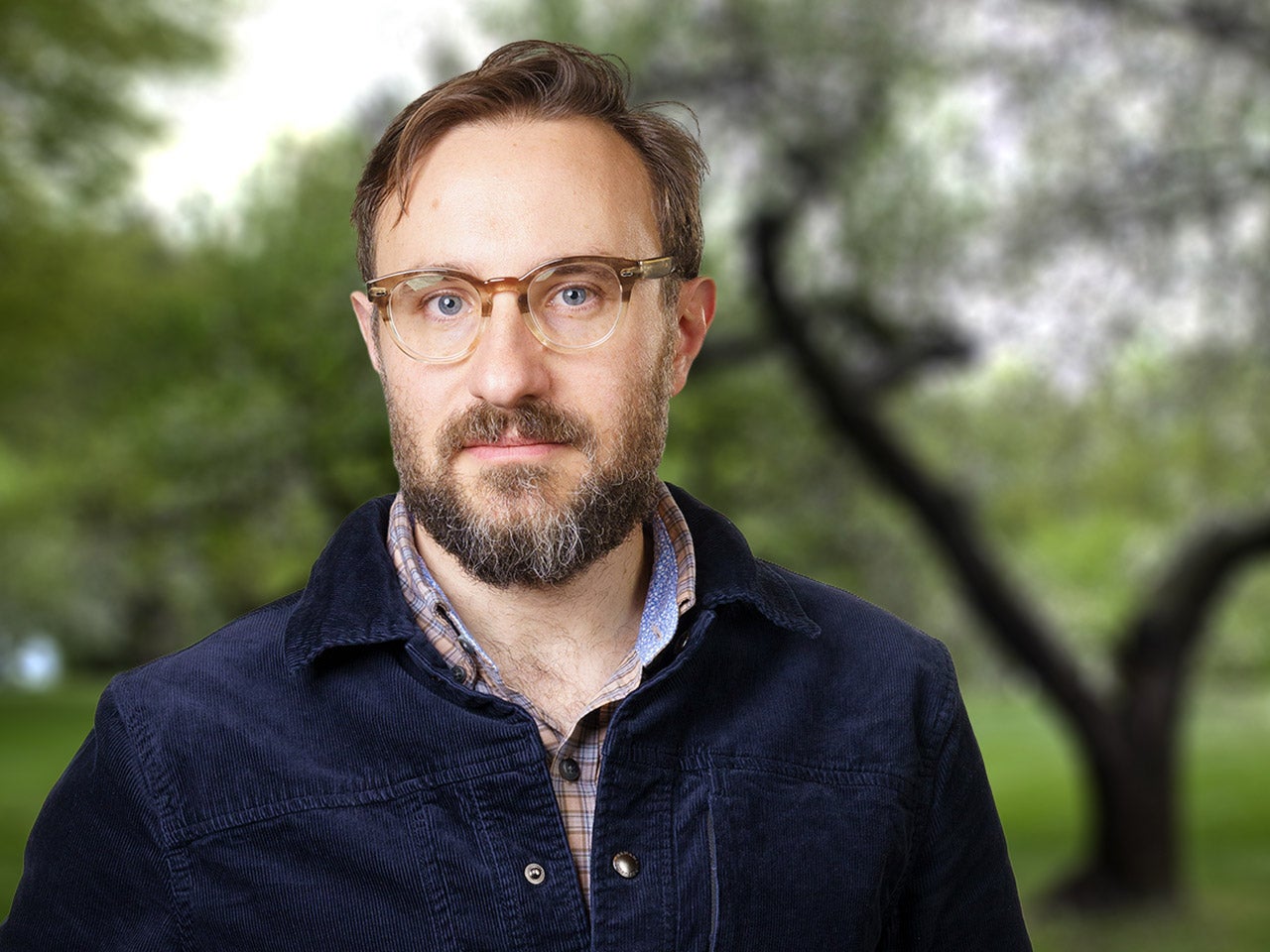
Justin Kinney
Associate Professor
Cancer Center Program Co-Leader
Ph.D., Princeton University, 2008
jkinney@cshl.edu | 516-367-5230
Research in the Kinney Lab combines mathematical theory, machine learning, and experiments in an effort to illuminate how cells control their genes. These efforts are advancing the fundamental understanding of biology and biophysics, as well as accelerating the discovery of new treatments for cancer and other diseases.
Dr. Kinney completed his Ph.D. in Physics at Princeton University in 2008 and began his term as a Quantitative Biology Fellow at CSHL in 2010. His research focuses on developing next-generation DNA sequencing as a tool for dissecting the biophysical mechanisms of gene regulation. As a graduate student, Kinney co-invented a widely used technique now known as the massively parallel reporter assay (MPRA). Kinney and colleagues further showed how, using ideas from information theory, such experiments could be used to infer quantitative biophysical models for how cells regulate gene expression. The Kinney lab continues to leverage a tightly knit combination of mathematical theory, machine learning, and experiments in order to illuminate the biophysics of gene regulation in two diverse contexts: bacterial transcriptional regulation and alternative mRNA splicing in humans. This latter context is highly relevant to understanding and treating human diseases like Spinal Muscular Atrophy and Cancer. The Kinney lab also develops algorithms and software for the analysis of MPRAs and other multiplex assays of variant effect (MAVEs).
SQUID pries open AI black box
June 21, 2024
CSHL’s Koo and Kinney labs have built a tool to suss out how AI analyzes the genome. What sets it apart? Decades of quantitative genetics knowledge.
Why some RNA drugs work better than others
March 6, 2024
CSHL’s Justin Kinney and Spinraza inventor Adrian Krainer tested the newly approved SMA treatment, risdiplam, and another RNA therapeutic, branaplam.
President’s essay: Bringing bold visions to life
May 26, 2023
CSHL President & CEO Bruce Stillman sees the Laboratory as a global hub for scientific expertise and a powerful launchpad for early-career scientists.
The rise of RNA therapeutics
October 14, 2021
RNA has been making waves as a new approach to prevent or treat diseases, including COVID-19 and spinal muscular atrophy.
Calculating the path of cancer
October 4, 2021
A new mathematical approach is helping cancer researchers at CSHL determine how mutations lead to different behaviors in cancerous cells.
Finally, machine learning interprets gene regulation clearly
December 26, 2019
Machine learning and a new kind of easily-interpretable artificial neural network is helping scientists make sense of crucial gene regulation.
Peter Koo wants to understand how machines learn biology
September 20, 2019
Dr. Peter Koo joins the CSHL faculty as an assistant professor. His focus is on exploring how artificial intelligence integrates with biology and genomics.
Event: Public Lecture: Seeing With Sequencing
August 8, 2019
Come hear from three quantitative biologists as they discuss how they see with sequencing to solve mysteries ranging from the genetics of evolution.
Seeing with sequencing—A public lecture with three CSHL experts
April 19, 2019
Quantitative biologists discuss how physics, modern computing power, and a new perspective on biology can make sense of our complex genomes.
The big problem of small data: A new approach
October 18, 2018
You’ve heard of “big data” but what about small? Researches have crafted a modern approach that could solve a decades-old problem in statistics.
All Publications
Interpreting cis-regulatory mechanisms from genomic deep neural networks using surrogate models
Jun 2024 | Nature Machine Intelligence | 6(6):701-713
Seitz, E, McCandlish, D, Kinney, J, Koo, P
Gauge fixing for sequence-function relationships
13 May 2024 | bioRxiv
Posfai, Anna, Zhou, Juannan, McCandlish, David, Kinney, Justin
Symmetry, gauge freedoms, and the interpretability of sequence-function relationships
13 May 2024 | bioRxiv
Posfai, Anna, McCandlish, David, Kinney, Justin
Specificity, synergy, and mechanisms of splice-modifying drugs
29 Feb 2024 | Nature Communications | 15(1):1880
Ishigami, Yuma, Wong, Mandy, Martí-Gómez, Carlos, Ayaz, Andalus, Kooshkbaghi, Mahdi, Hanson, Sonya, McCandlish, David, Krainer, Adrian, Kinney, Justin
Interpreting cis -regulatory mechanisms from genomic deep neural networks using surrogate models
16 Nov 2023 | bioRxiv
Seitz, Evan, McCandlish, David, Kinney, Justin, Koo, Peter
Specificity, cooperativity, synergy, and mechanisms of splice-modifying drugs
10 Feb 2023 | Biophysical Journal | 122(3S1):271a
Ishigami, Y, Wong, M, Aldaravi, C, Kooshkbaghi, M, Ayaz, A, McCandlish, D, Krainer, A, Kinney, J
Specificity, synergy, and mechanisms of splice-modifying drugs
31 Dec 2022 | bioRxiv
Ishigami, Yuma, Wong, Mandy, Martí-Gómez, Carlos, Ayaz, Andalus, Kooshkbaghi, Mahdi, Hanson, Sonya, McCandlish, David, Krainer, Adrian, Kinney, Justin
Higher-order epistasis and phenotypic prediction
27 Sep 2022 | Proceedings of the National Academy of Sciences of the United States of America | 119(39):e2204233119
Zhou, Juannan, Wong, Mandy, Chen, Wei-Chia, Krainer, Adrian, Kinney, Justin, McCandlish, David
Structural and mechanistic basis of σ-dependent transcriptional pausing
7 Jun 2022 | Proceedings of the National Academy of Sciences of the United States of America | 119(23):e2201301119
Pukhrambam, Chirangini, Molodtsov, Vadim, Kooshkbaghi, Mahdi, Tareen, Ammar, Vu, Hoa, Skalenko, Kyle, Su, Min, Yin, Zhou, Winkelman, Jared, Kinney, Justin, Ebright, Richard, Nickels, Bryce
MAVE-NN: learning genotype-phenotype maps from multiplex assays of variant effect
15 Apr 2022 | Genome Biology | 23(1):98
Tareen, Ammar, Kooshkbaghi, Mahdi, Posfai, Anna, Ireland, William, McCandlish, David, Kinney, Justin