Genes make up only a small fraction of the human genome. Between them are wide sequences of DNA that direct cells when, where, and how much each gene should be used. These biological instruction manuals are known as regulatory motifs. If that sounds complex, well, it is.
The instructions for gene regulation are written in a complicated code, and scientists have turned to artificial intelligence to crack it. To learn the rules of DNA regulation, they’re using deep neural networks (DNNs), which excel at finding patterns in large datasets. DNNs are at the core of popular AI tools like ChatGPT. Thanks to a new tool developed by Cold Spring Harbor Laboratory Assistant Professor Peter Koo, genome-analyzing DNNs can now be trained with far more data than can be obtained through experiments alone. Koo says:
“With DNNs, the mantra is the more data, the better. We really need these models to see a diversity of genomes so they can learn robust motif signals. But in some situations, the biology itself is the limiting factor, because we can’t generate more data than exists inside the cell.”
If an AI learns from too few examples, it may misinterpret how a regulatory motif impacts gene function. The problem is that some motifs are uncommon. Very few examples are found in nature.
To overcome this limitation, Koo and his colleagues developed EvoAug—a new method of augmenting the data used to train DNNs. EvoAug was inspired by a dataset hiding in plain sight—evolution. The process begins by generating artificial DNA sequences that nearly match real sequences found in cells. The sequences are tweaked in the same way genetic mutations have naturally altered the genome during evolution.
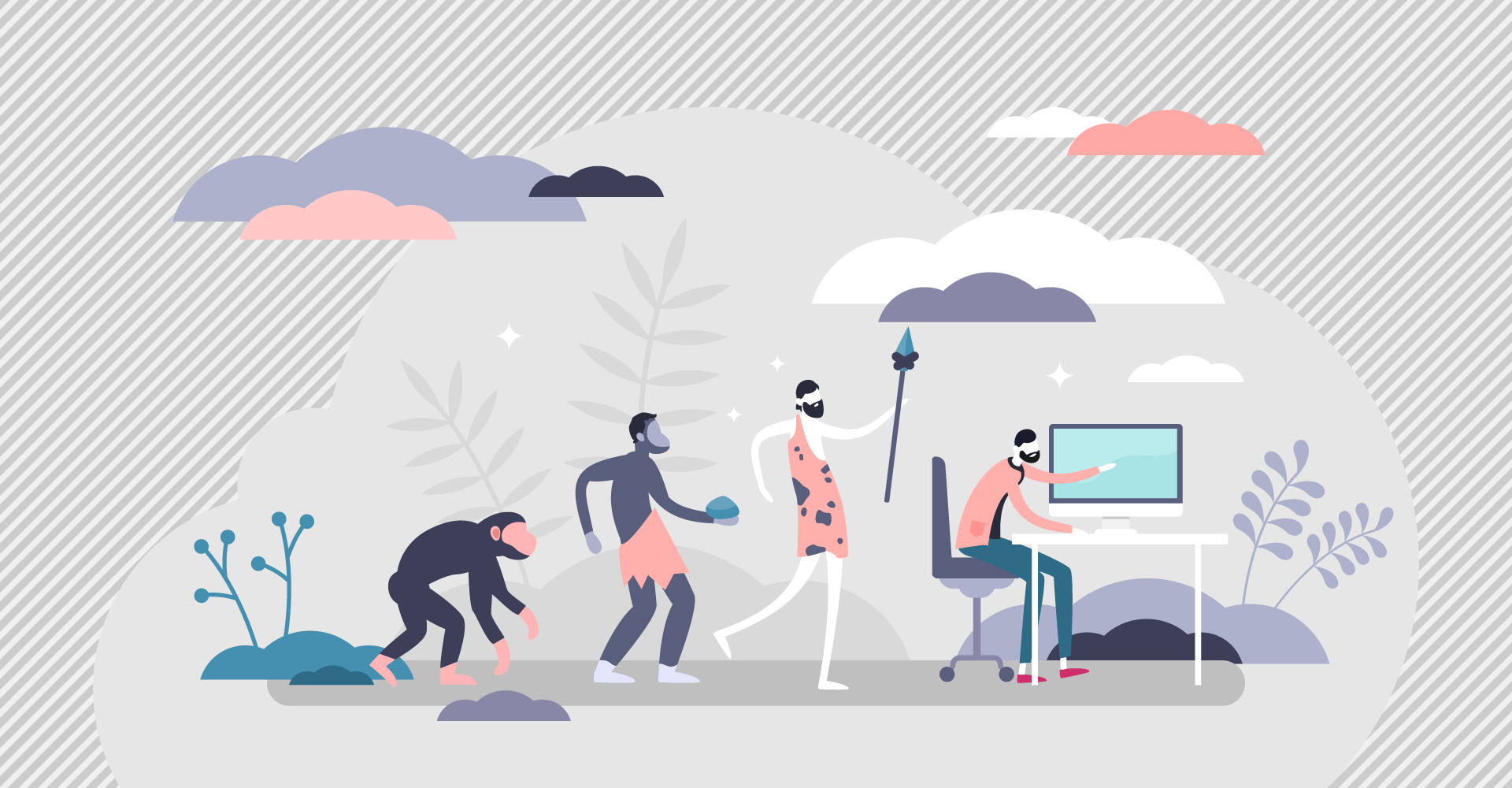
Next, the models are trained to recognize regulatory motifs using the new sequences, with one key assumption. It’s assumed the vast majority of tweaks will not disrupt the sequences’ function. Koo compares augmenting the data in this way to training image-recognition software with mirror images of the same cat. The computer learns that a backward cat pic is still a cat pic.
The reality, Koo says, is that some DNA changes do disrupt function. So, EvoAug includes a second training step using only real biological data. This guides the model “back to the biological reality of the dataset,” Koo explains.
Koo’s team found that models trained with EvoAug perform better than those trained on biological data alone. As a result, scientists could soon get a better read of the regulatory DNA that write the rules of life itself. Ultimately, this could someday provide a whole new understanding of human health.
Written by: Jennifer Michalowski, Science Writer | publicaffairs@cshl.edu | 516-367-8455
Funding
National Institutes of Health, Simons Center for Quantitative Biology at Cold Spring Harbor Laboratory
Citation
Lee, N., et al., “EvoAug: improving generalization and interpretability of genomic deep neural networks with evolution-inspired data augmentations”, Genome Biology, May 4, 2023. DOI: 10.1186/s13059-023-02941-w
Principal Investigator
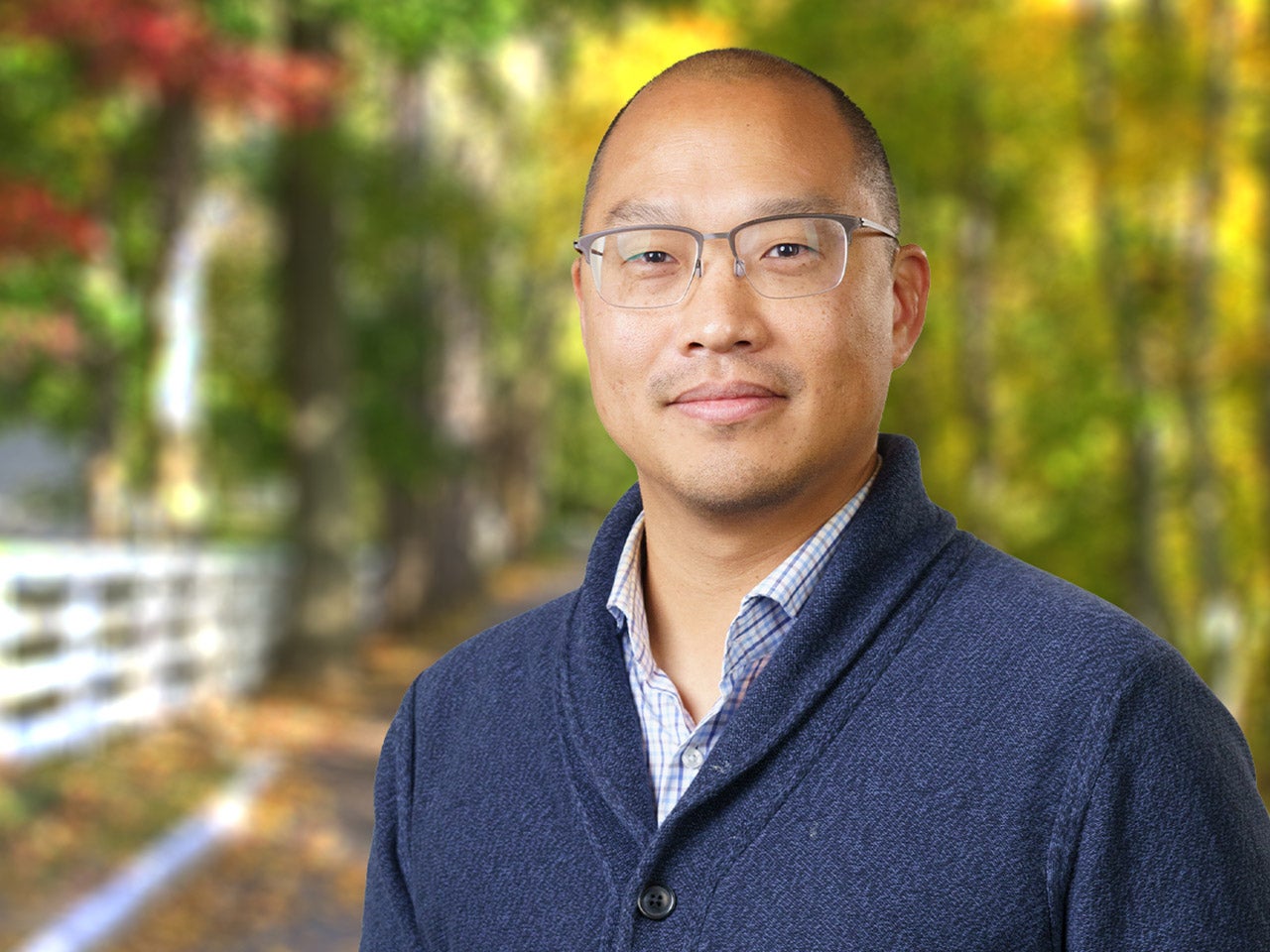
Peter Koo
Associate Professor
Cancer Center Member
Ph.D., Yale University, 2015