When OpenAI released ChatGPT in November 2022, artificial intelligence quickly hit the mainstream and became a global cultural phenomenon. The chatbot dominated headlines as it authored news articles, passed the bar exam, drafted lesson plans for teachers, and even wrote code. ChatGPT became the fastest-growing consumer application ever.
In the months following its launch, companies scrambled to create competing large language models. This started what many consider an AI “arms race.” Now, the technology is everywhere you look. Yet, artificial intelligence is much bigger than ChatGPT and the other generative AI systems that have taken the world by storm. Models that use AI have been working behind the scenes for years in fields ranging from cybersecurity to science and medicine.
At Cold Spring Harbor Laboratory (CSHL), researchers work to unlock AI’s full potential by combining AI and neuroscience. They hope one day smarter models will lead to advancements that benefit everyone, not just the lucky few.
What is AI? And what isn’t it?
Artificial intelligence, or AI, is a scientific field that uses computers to solve problems that typically require human intelligence. Large language models like ChatGPT are trained on vast amounts of data scraped from all corners of the internet, including Web pages, digitized books, Wikipedia articles, and posts on public forums like Reddit.
These chatbots use “neural networks,” or algorithms inspired by how the brain works, to process new data and detect patterns without human input. “Most of modern AI is actually an outgrowth of neuroscience,” argues CSHL Professor Anthony Zador.
CSHL NeuroAI Scholar Kyle Daruwalla talks about making AI more energy-efficient and accessible for all.
Because large language models have access to such massive amounts of information, they can learn and act in ways that meet and sometimes even surpass our own abilities.
“We do have chatbots right now that have very convincing and realistic conversations with humans,” says Kyle Daruwalla, a NeuroAI scholar at CSHL. “But it’s skin deep,” he adds. “There’s no depth behind the words that are said.”
In other words, AI models do not have independent thoughts or feelings and do not truly understand meaning. However, they are extremely good at pretending they do. Some are so good, they’ve persuaded users they’re actually sentient. So, how will we know if one ever does possess human levels of intelligence? And will this happen anytime soon?
Move over, Turing
In 1950, mathematician Alan Turing developed a test to determine whether computers could behave intelligently. He proposed a scenario in which a human judge would converse with both an AI model and another human. If the judge could not tell the difference between the two, that meant the model possessed human-like intelligence. Turing didn’t specify many details about how the test would be carried out. So, scientists disagree over whether or not certain AI chatbots have passed. Many disagree with the test entirely. They say it doesn’t truly indicate an AI model’s ability to “think.” Additionally, the test assumes that language represents peak human intelligence, Zador and his colleagues say.
“It doesn’t account for our abilities to interact with and reason about the physical world,” Zador says. So, he and his colleagues have called for a new “embodied” Turing test grounded in principles of NeuroAI, a field at the intersection of neuroscience and AI. “NeuroAI is based on the idea that a better understanding of the brain will reveal the ingredients of intelligence and eventually lead to human-level AI,” Zador explains.
The new test would pit AI animal models against their real-world counterparts, assessing how well each interacts with its environment. The test shifts focus away from AI models that play games and use language, which are capabilities that are especially well-developed in or unique to humans. Instead, it assesses abilities shared by all animals, including sensorimotor skills and interactions with unpredictable environments.
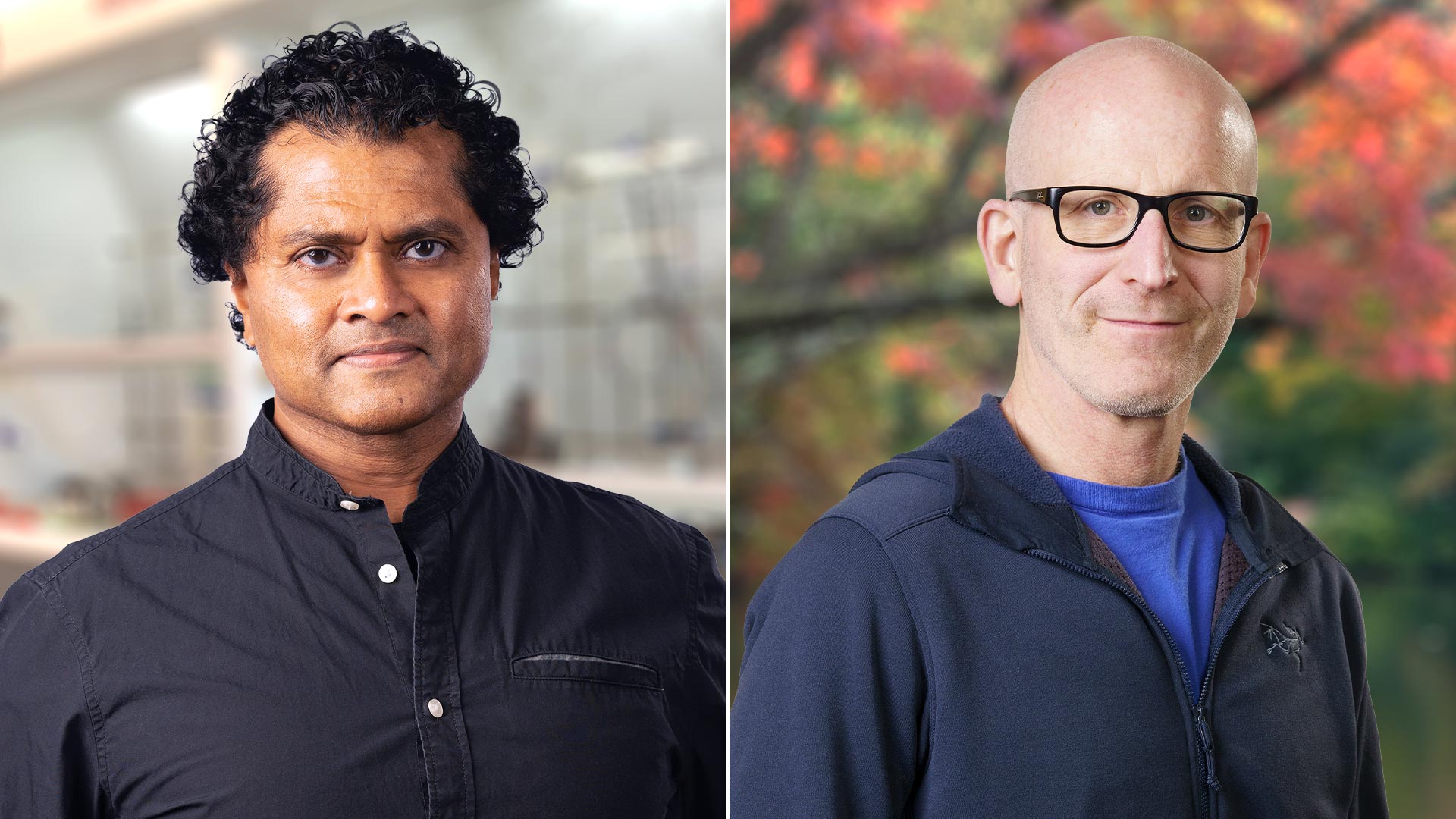
Think about walking down a crowded city sidewalk. AI isn’t there yet. In fact, it isn’t able to walk at all. The robots you may have seen running through obstacle courses? They’re programmed to do that. They don’t learn it. Therefore, they aren’t AI.
Building systems that can pass the embodied Turing test could accelerate the next generation of AI, according to Zador. Yet, to reach this next generation, Zador and his colleagues believe we must bridge the gap between neuroscience and AI. Many of the cutting-edge AI systems we use today were inspired by old neuroscience from the 1950s and ‘60s. Though scientists have made more recent breakthroughs, these advancements have yet to translate over to AI.
To combine these two fields, resources will need to be allocated into three main areas: training researchers equally in neuroscience and AI, creating an open and shared platform to build and test AI systems, and investing in fundamental research that will allow us to define the ingredients of intelligence.
Using AI to map the mind
Not only can neuroscience help us build better AI, but AI may help us better understand neuroscience, too. CSHL Professor Partha Mitra uses AI to uncover more about our brain circuitry.
“We want to understand how we think, how we feel,” Mitra says. “These are philosophical questions that have been around for thousands of years. And we have made significant progress in the 20th century, but we still lack essential data sets.”
In the old days, studying neural circuits required scientists to look carefully at sections of the brain under a microscope. Yet, as technology improved and computers became able to store much more data, this method became obsolete. Scientists now believe the human brain has around 100 billion neurons, each of which talks with a thousand other neurons.
“The data from just one human brain at a light microscopic scale is about a petabyte, which is very large,” Mitra says. “To study that, we need assistance from the computer. We are trying to automate the analysis of brain circuitry from microscopic images so that we can ultimately reach our goal of understanding how these circuits work.”
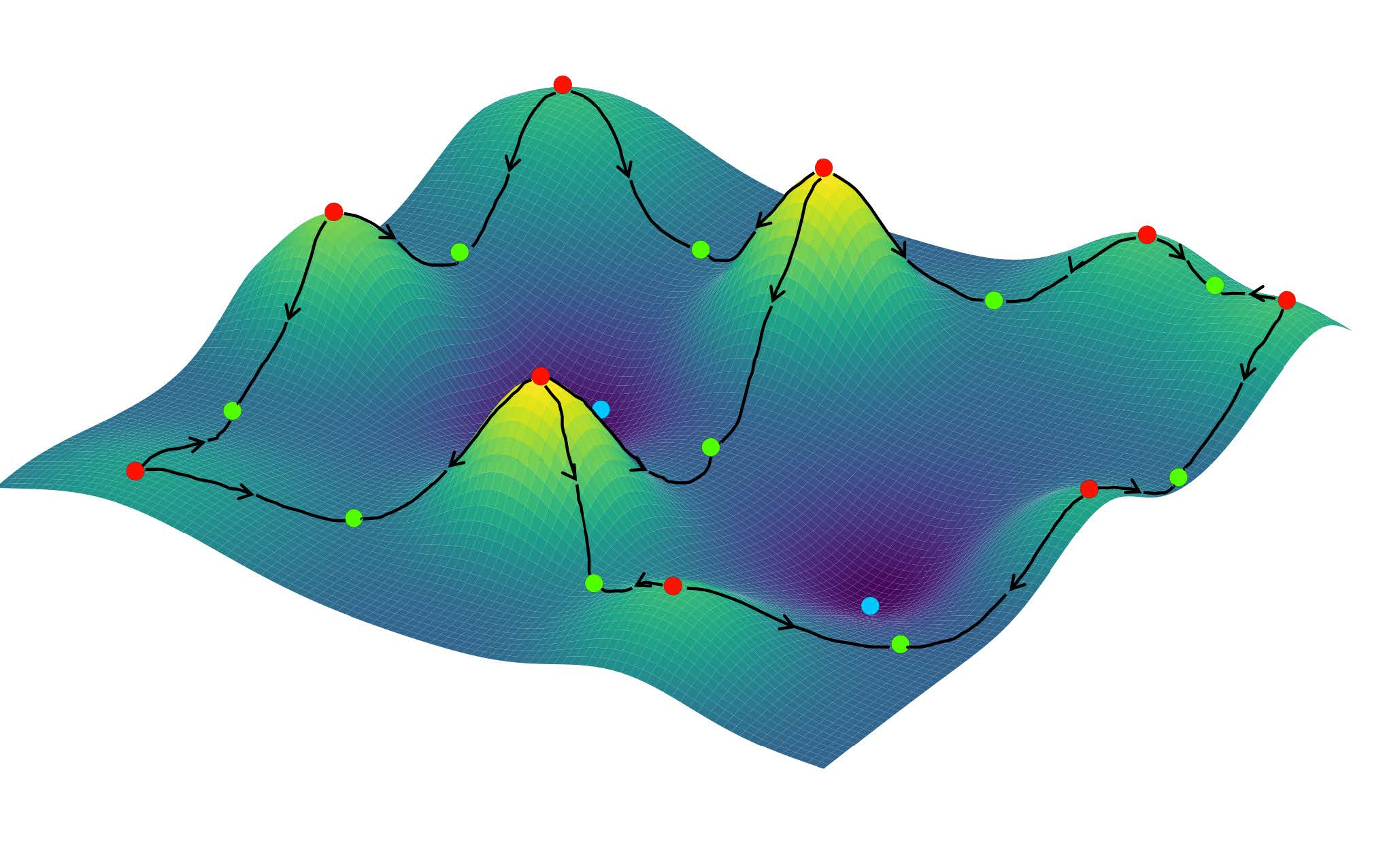
Mitra and his colleagues have developed a method of tracing neurons and their connections more efficiently, using a combination of machine-learning techniques and a form of mathematics called topological data analysis. Topological data analysis looks at the specific shape of data in a way that emphasizes connectivity. This method allows the Mitra lab to teach algorithms to detect specific nerve cells and fibers more accurately without as much human oversight.
Not only could knowing how our brain circuitry works lead to better AI models. It could have psychiatric applications, too. Eventually, we may be able to pinpoint differences in the brain circuits of people living with neurological disorders or mental health conditions.
“If you don’t know what’s different, one can’t really start addressing it,” Mitra says. “And you may or may not want to address that difference.”
To ensure their findings will have a broad impact on society, the researchers are making their code available for others to use on their own data.
AI in the future
Artificial intelligence has revolutionized the speed and way in which we process data. Yet, like any emerging technology, AI has sparked widespread fears over how it could be used in the future. Some of these fears are warranted, especially those over the spread of disinformation and job loss, Zador says. However, the hype over AI becoming self-aware isn’t a pressing fear—at least not yet.
Daruwalla views AI in the same light as the internet. It will certainly make dramatic changes in our lives and exacerbate existing problems, but it should ultimately be a force for good.
CSHL Assistant Professor Peter Koo is training AI to search the genome for potential breast cancer risk factors.
In fact, a better understanding of the brain could be just the beginning. Right now, there are CSHL scientists using AI to analyze cancer and other diseases. Their findings could help inspire lab experiments that point to new drug targets and therapeutic strategies.
“I think the best use of it is for scientific research, for drug discovery,” Daruwalla says. “The output of that is objectively going to make all of our lives better.”
Written by: Margaret Osborne, Science Writer | publicaffairs@cshl.edu | 516-367-8455