A couple years ago, the pioneering geneticist Craig Venter went viral by failing to appear in a video clip.
The presenter of a TED talk—a biologist named Riccardo Sabbatini—welcomed Venter onto the stage to explain the staggering amount of information in the human genetic code. As people began to applaud, five assistants emerged from the wings, wheeling carts containing 175 encyclopedia-size books onto the stage. Venter the actual scientist wouldn’t be coming, Sabbatini explained, but inside those books were 262,000 pages containing the 3 billion DNA letters of the eminent man’s genome—“the visual perception of the code of life.” The audience gasped when Sabbatini cracked open one of the books: Even stretched out over 175 volumes, the letters had to be written so small that each page resembled a black square filled with dots.
That is the great challenge facing today’s genetic sleuths. Nearly two decades ago, the Human Genome Project created the first complete map of our genes, promising grand new insights into disease and treatments. But it has been exceedingly difficult to make meaningful sense out of that flood of data. Now, software-literate computational biologists are harnessing advances in machine learning and data mining to begin to do what the human mind alone could not. They are running comparisons between individuals and between species, seeking out meaningful patterns. They are identifying which portions of the genome, when mutated, are most likely to cause disease. And some have begun applying new analytical tools to saving lives.
“We are starting to see the application of machine learning approaches in interpreting the genetic variations in human patients,” says David Goldstein, founding director of the Institute for Genomic Medicine at the Columbia University Medical Center.
It’s an approach pioneered at the University of California at Santa Cruz (UCSC) in the early 2000s, with much of the key work done by a young graduate student with a background in biology and computers named Adam Siepel. By then geneticists had already sequenced the much smaller genetic code of one other species, the fugu fish. Soon after, they finished the human genome, quickly followed by the mouse and rat genomes. Researchers lined up the various codes for comparison, hoping to identify the most important genetic regions, says Jim Kent, the UCSC research scientist who had lead the effort to stitch together that first complete human genome.
After Kent and his colleagues teed up the project, it fell to Siepel to design a program that transformed the cross-species comparison into a searchable database. The goal was to let researchers around the globe type in specific genetic sequences and receive a result predicting how likely that sequence was to have some functional importance. Kent and his team reasoned that if a certain chunk of DNA appears nearly the same across divergent species—if it is “highly conserved,” in genetics terminology—it must crucial for life.
“We were searching for things that looked like they had been under very strong selection to remain unchanged for millions of years, because if they were that conserved by evolution, they were likely to be important,” says Siepel, who is now the chair of the Simons Center for Quantitative Biology at Cold Spring Harbor Laboratory, a private, not-for-profit scientific research institution on Long Island.
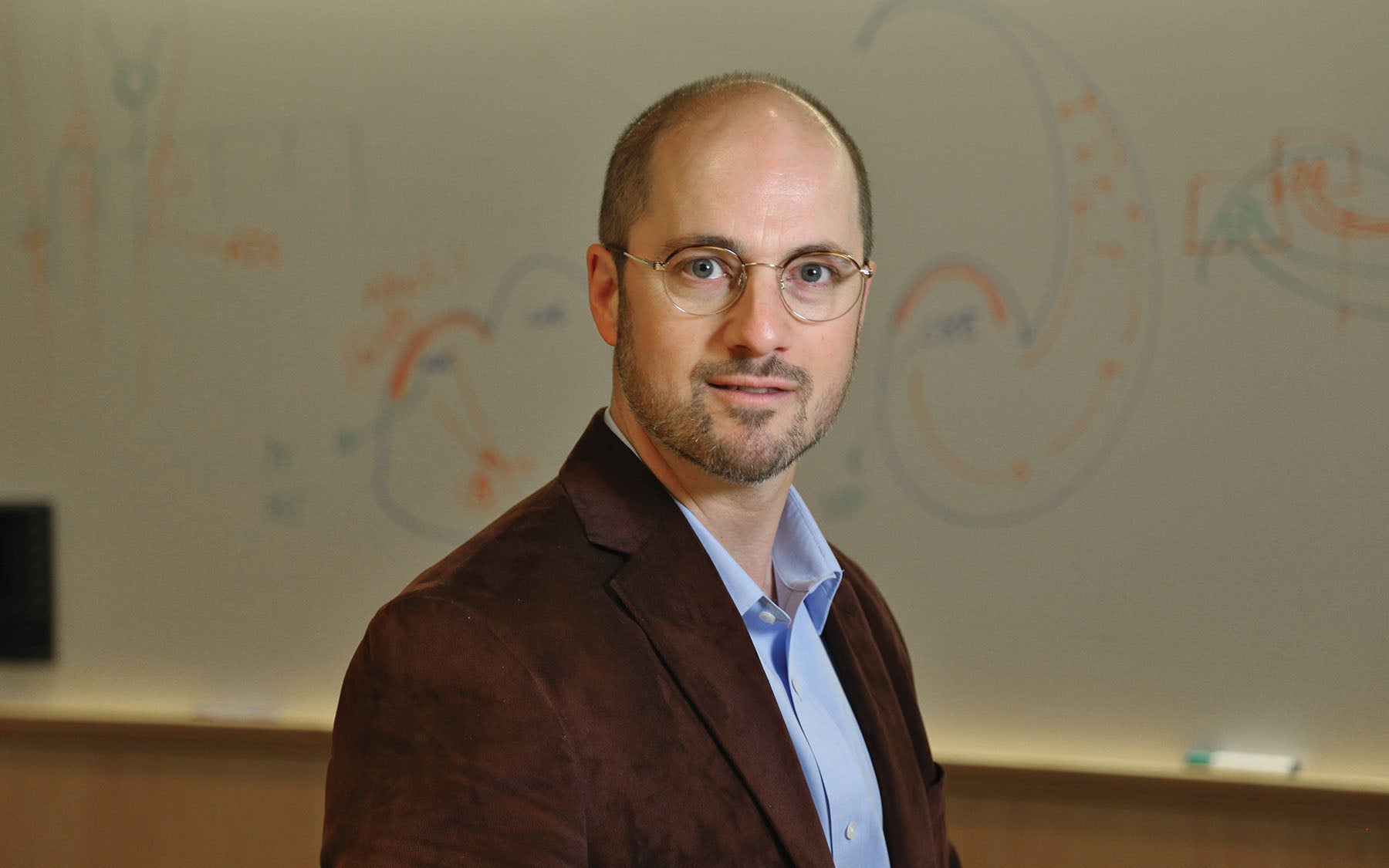
By running genetic data through pattern-recognition computer programs, Siepel developed mathematical models of evolution that identified genetic sequences likely to be essential to survival. Those models were incorporated into the UCSC Genome Browser, a public web site containing a copy of the human genome and a variety of other tools for making sense of it. The site also included visualization tools and databases that allow geneticists to type in a specific gene and pull up annotations explaining what is currently known about its role and function. Much to Siepel’s surprise, he says, his track on the Genome Browser “took off like wildfire.”
“Today, there are upwards of several hundred thousand biomedical researchers who use that information,” says Benedict Paten, director of the Computational Genomics Laboratory and an associate director of the UC Santa Cruz Genomics Institute, referring specifically to Siepel’s conserved-code database. “It was hugely impactful.”
Siepel has since continued to build on that approach. In a 2015 paper in Nature Genetics, he unveiled a new computational method that analyzes variations within the whole human genome, rather than between species, to assign what he calls a “fitness consequence (fitCons) score,” to estimate the probability that a specific mutation in the vast genetic sequence will cause problems. As an example of the power of small changes in the genome, an error of just two genetic letters on human chromosome 14 can cause a person to have cystic fibrosis—as yet, with no hope of a cure.
“The higher the fitCons score, the rarer the mutation in the human population—implying those who have one are unlikely to survive long enough to pass that mutation on to their offspring,” Siepel says. In 2017, he introduced a related computational method called LINSIGHT, aimed at making it easier to predict the impact of mutations that act indirectly: they don’t affect the genes that direct the creation of vital proteins in the body, but rather they change parts of the DNA that modulate the action of those protein-coding genes.
In parallel, Goldstein and his colleagues have pioneered a data-mining method that examines the rate of variability within human populations and the extent to which mutations seem to have no deleterious effect. Their results make it easier to rule out certain mutations as the cause of disease. They call their approach “intolerance scoring.” It works by calculating the rate of variation across human populations. On average, each person will be born with around 100 new mutations, which should be scattered randomly through the genome. By analyzing DNA from a large number of people, Goldstein can then see how many mutations actually show up in a specific sequence of DNA and how frequently they are passed on. In this way, he and his team can infer how many mutations that sequence will “tolerate” before it has a negative impact on health, and selection pressure begins to weed it out.
If a given sequence has far fewer mutations than expected in the overall population, Goldstein would flag it a low tolerance score, meaning that it deserves closer study. The lower the tolerance score, the more likely that sequence is to be one of the sources of trouble for a patient with a mysterious genetic disease.
These various tools work together to assist doctors in narrowing down which of a patient’s many unusual DNA features could be causing health problems. “It’s not unusual to come up with thousands of reasonable candidates, of which a patient might have had 50 or 100 that are quite plausible,” UCSC’s Kent explains. Tools like Siepel’s fitness consequence could take that number down by a fact of five, and Goldstein’s tolerance score could reduce it further still.
Far greater benefits from computational biology lie ahead. Soon it might be possible to feed a patient’s DNA sequence into a computer program and, using artificial intelligence, receive an instant, automated diagnosis and an analysis of which portions of the genome are causing the disease. Once doctors have identified the specific biological mechanisms causing these diseases, they might be able to synthesize drugs tailored to that patient’s specific genome or, perhaps, even correct the faulty section of DNA using a gene-editing tool known as CRISPR. But hacking the genome is already helping doctors save lives.
Goldstein cites the example of a four-year-old girl with a progressive neurological illness that had weakened her upper body to the point where she could no longer lift her arms or hold up her head. Her doctors were largely stumped, and feared she would die. But by applying the tools of computational biology to supplement his own medical expertise and experience, Goldstein was able to pick up all the mutations specific to her DNA and to identify the two most likely to be causing the girl’s problems. Eventually, by considering her symptoms as well, he and his team homed in on a mutation to a gene important in the body’s ability to absorb vitamin B2—a disease so rare it is found in about 60 people worldwide. Within a few months of taking oral vitamin supplements, the girl was able to visit her doctors to thank them by running up and down the hallway, giving them all high-fives.
In this case, curing the patient required a mixture of old-fashioned medical sleuthing and cutting-edge machine learning. That’s the way medicine is likely to remain for a while—but with the gene-hacking machines lending more and more of a hand. “There’s more excitement about what machine learning approaches might do in the future as opposed to the big impact they make today. The reality is that most serious applications of genomics in a medical context still require expert judgement,” Goldstein says.
The reason, he explains, comes back to the 3 billion DNA letters (or the 262,000 printed pages) of the human genome. Machine learning works well when it is applied to a dataset that is already well explored. For instance, the computer scientists trying to design self-driving cars can draw on detailed knowledge of the rules of the road, driver behaviors, common obstacles, causes of accidents, and so on. “Artificial intelligence can start to drive cars pretty well because the space of what happens when you drive a car is reasonably explored, but straight AI approaches for genome interpretation don’t work well right now,” Goldstein notes. And even with that advantage, fully autonomous vehicles are not yet good enough to set loose in the world.
Siepel’s LINSIGHT project will be important in filling in information about the whole of the human genome, not just the 1 percent that has a well-understood biological function. Meanwhile, intolerance scoring will help by identifying the parts of the human genome that are most likely to be associated with disease—based entirely on computer-driven data analysis, without any human assumptions or biases in the mix. Goldstein thinks that scientists will need to compile and compare genes from millions of people before an AI can usefully analyze your whole genetic makeup, identify problems, and point to specific treatments.
“So for those of us who consider ourselves informed experts in the interpretation of genomic variation, I think we still have jobs for at least five-plus years,” he says. After that, though, an even greater revolution awaits.
For more about the science underway at Cold Spring Harbor Laboratory and the rest of the world, please visit the new Biology+Beyond channel on Nautilus.
Written by: Adam Piore, Nautilus Writer