Talking about your bad day at work could lead to great solutions. Cold Spring Harbor Laboratory (CSHL) Associate Professor Saket Navlakha and his wife, Dr. Sejal Morjaria, an infectious disease physician at Memorial Sloan Kettering Cancer Center (MSK), found a way to predict COVID-19 severity in cancer patients. The computational tool they developed prevents unnecessary expensive testing and improves patient care.

Morjaria says, “Generally, I have good intuition for how patients will progress.” However, that intuition failed her when confronted with COVID-19. She says:
“When the pandemic first hit, we had a hard time understanding and predicting which patients were going to have severe COVID. People were ordering a slew of labs, and a lot of times, there were unnecessary lab tests.”
Navlakha joined CSHL in 2019. He uses computer science to understand biological processes. Morjaria wondered if her husband could help:
“So I came home and I would tell him, ‘Saket, it would be great if we could come up with a methodology to figure out, using machine-learning, which patients are going to go on to develop severe COVID versus not.’”
The team collected 267 variables from cancer patients diagnosed with COVID-19. The variables ranged from age and sex to cancer type, most recent treatments, and laboratory results. They trained a machine-learning computer program to classify patients into three groups. Those who will require high levels of oxygen through a ventilator:
- immediately
- after a few days
- not at all
The researchers found approximately 50 variables that contributed most to the outcome prediction. Their method had an accuracy rate of 70–85%, and it performed especially well for patients that would require immediate ventilation. More generally, the tool can help tease apart interactions between multiple risk factors that might not be apparent, even to those with trained eyes. The program also prevents over-testing, which Morjaria knows will “spare patients unnecessary massive hospital costs.”
Navlakha believes this work would not have been possible without close collaboration with his wife and other MSK clinician-scientists, including Rocio-Perez Johnston and Ying Taur. He says:
“Sejal and I talk about better ways to integrate what she’s experiencing on the bedside versus what we can analyze and do computationally. As someone who’s never worked with clinical data, if I were to try to have done this without Sejal’s guidance, I would have made tons of mistakes, it would have just been a total disaster and totally unusable.”
Navlakha and Morjaria hope their work will inspire more physicians and computer scientists to work together and create innovative clinical solutions for complex diseases.
Written by: Jasmine Lee, Content Developer/Communicator | publicaffairs@cshl.edu | 516-367-8845
Funding
Simons Center for Quantitative Biology at Cold Spring Harbor Laboratory
Citation
Navlakha, S., et al., “Projecting COVID-19 disease severity in cancer patients using purposefully-designed machine learning”, BMC Infectious Diseases, May 4, 2021. DOI: 10.1186/s12879-021-06038-2
Principal Investigator
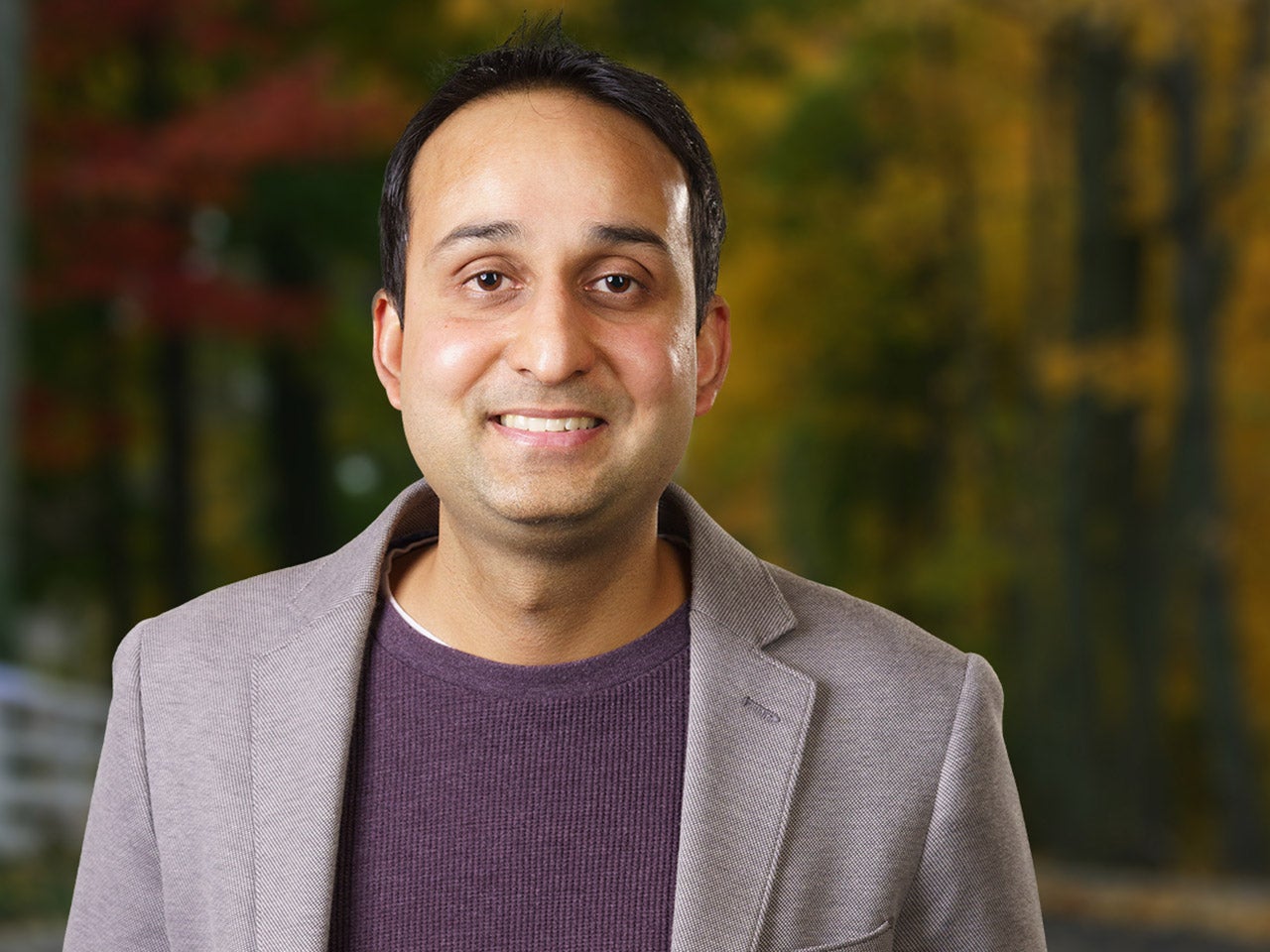
Saket Navlakha
Associate Professor
Ph.D., University of Maryland College Park, 2011